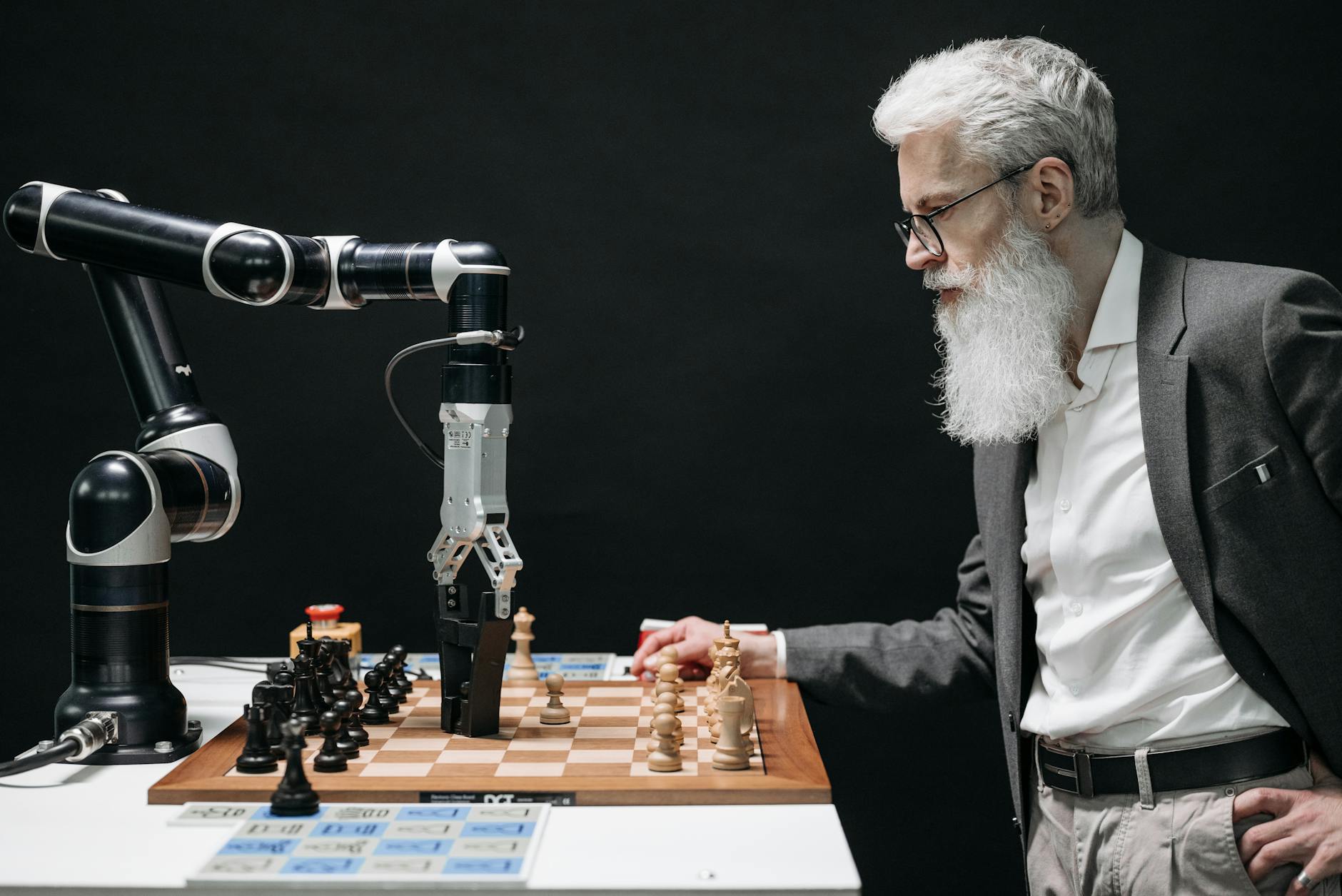
Are you ready for the AI revolution? 🚀 Brace yourself, because the tech giants are locked in an intense battle for artificial intelligence supremacy. From Silicon Valley to Seattle, and across the globe, companies are pouring billions into AI research and development, each vying to be the one who cracks the code to the next big breakthrough.
But this isn’t just about bragging rights or stock prices. The AI arms race among big tech has far-reaching implications for all of us. It’s shaping the future of how we work, communicate, and even think. As these tech behemoths push the boundaries of what’s possible, they’re not just competing with each other—they’re racing against time, ethical concerns, and regulatory hurdles.
In this deep dive, we’ll explore the major players in this high-stakes game, the cutting-edge technologies fueling the competition, and the scramble for top AI talent. We’ll also examine the ethical minefields and regulatory challenges that lie ahead, the impact on the products and services we use daily, and what the future might hold in this relentless pursuit of AI dominance. Buckle up—it’s going to be an exhilarating ride through the world of artificial intelligence! 🤖💡
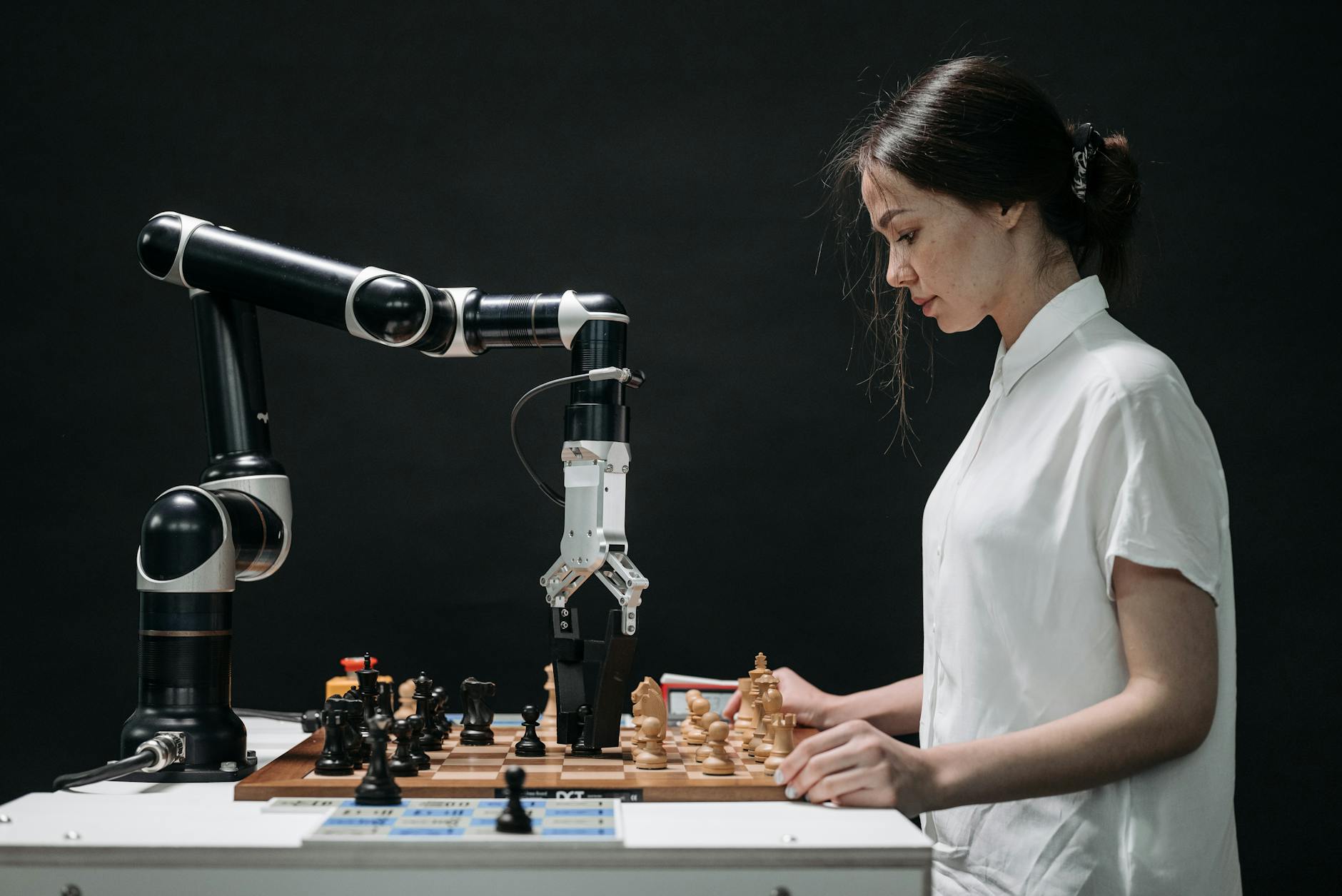
The Major Players in the AI Race
The artificial intelligence (AI) arms race among big tech companies has intensified in recent years, with major players investing billions of dollars and dedicating vast resources to gain a competitive edge in this transformative technology. This section will delve into the AI initiatives, breakthroughs, and strategic investments of four key players: Google, Microsoft, Meta, and Amazon. These tech giants are at the forefront of AI innovation, pushing the boundaries of what’s possible and shaping the future of technology.
A. Google’s AI initiatives and breakthroughs
Google, a subsidiary of Alphabet Inc., has long been a pioneer in AI research and development. The company’s commitment to AI is evident in its numerous initiatives and groundbreaking achievements.
1. DeepMind Acquisition and Advancements
One of Google’s most significant moves in the AI space was the acquisition of DeepMind in 2014 for $500 million. This strategic investment has paid off handsomely, with DeepMind making remarkable strides in AI research and practical applications.
- AlphaGo: In 2016, DeepMind’s AlphaGo program defeated world champion Lee Sedol in the complex game of Go, a feat previously thought to be decades away. This achievement showcased the power of deep learning and reinforcement learning algorithms.
- AlphaFold: In 2020, DeepMind’s AlphaFold system made a major breakthrough in protein folding prediction, solving a 50-year-old grand challenge in biology. This advancement has significant implications for drug discovery and understanding diseases at the molecular level.
- Multitask AI: DeepMind has also made progress in developing AI systems that can perform multiple tasks, such as playing different Atari games or solving various mathematical problems, demonstrating the potential for more versatile AI applications.
2. Google Brain and TensorFlow
Google Brain, the company’s in-house AI research team, has been instrumental in advancing machine learning and deep learning technologies.
- TensorFlow: Developed by the Google Brain team, TensorFlow is an open-source machine learning framework that has become one of the most popular tools for AI development worldwide. Its widespread adoption has solidified Google’s influence in the AI community.
- Neural Machine Translation: Google Translate has benefited significantly from AI advancements, with the introduction of Neural Machine Translation in 2016 leading to more accurate and natural-sounding translations across multiple languages.
3. AI in Google Products
Google has integrated AI into many of its core products, enhancing user experience and functionality:
- Google Search: AI algorithms power Google’s search engine, improving results relevance, understanding user intent, and providing more accurate answers to complex queries.
- Google Assistant: The company’s virtual assistant leverages natural language processing and machine learning to understand and respond to voice commands, control smart home devices, and assist with various tasks.
- Google Photos: AI-powered image recognition and categorization have made Google Photos a powerful tool for organizing and searching personal photo collections.
- Google Maps: AI algorithms enhance route planning, traffic prediction, and location-based recommendations in Google Maps.
4. AI Hardware Development
Google has also invested in developing specialized hardware for AI computations:
- Tensor Processing Units (TPUs): These custom-built AI accelerator ASICs (Application-Specific Integrated Circuits) are designed to accelerate machine learning workloads. TPUs have given Google a significant advantage in training and running large-scale AI models.
5. AI Ethics and Responsible Development
Recognizing the ethical implications of AI, Google has taken steps to promote responsible AI development:
- AI Principles: In 2018, Google published a set of AI Principles to guide the ethical development and use of AI technologies.
- AI Ethics Board: The company established an external advisory board on AI ethics, although it was later dissolved due to controversies.
6. Google Cloud AI
Google Cloud offers a suite of AI and machine learning services to businesses and developers:
- AutoML: This service allows users to create custom machine learning models without extensive coding knowledge, democratizing AI development.
- Vision AI, Speech-to-Text, and Natural Language AI: These pre-trained models enable developers to integrate advanced AI capabilities into their applications easily.
7. Recent Developments and Future Directions
Google continues to push the boundaries of AI research and application:
- LaMDA and Generative AI: Google’s Language Model for Dialogue Applications (LaMDA) showcases advanced conversational AI capabilities. The company is also exploring generative AI for text, images, and other media.
- Quantum AI: Google is investing in quantum computing research, aiming to leverage quantum algorithms for AI applications, potentially leading to exponential speedups in certain computations.
- AI for Social Good: The company has launched initiatives like AI for Social Good, applying AI to address global challenges in areas such as healthcare, environmental conservation, and education.
Google AI Initiative | Description | Impact |
---|---|---|
DeepMind | AI research company acquired by Google | Breakthroughs in Go, protein folding, and multitask AI |
Google Brain | In-house AI research team | Development of TensorFlow and advancements in machine learning |
TensorFlow | Open-source machine learning framework | Widely adopted tool for AI development |
TPUs | Custom AI accelerator hardware | Enhanced performance for AI computations |
Google Cloud AI | Suite of AI services for businesses | Democratization of AI development and deployment |
AI Ethics Initiatives | Principles and advisory boards | Promotion of responsible AI development |
Quantum AI | Research into quantum computing for AI | Potential for exponential speedups in AI computations |
Google’s multifaceted approach to AI, combining cutting-edge research, hardware development, ethical considerations, and practical applications, has positioned the company as a leader in the AI arms race. Its continuous innovation and strategic investments in AI technologies have not only enhanced its core products but also pushed the boundaries of what’s possible in artificial intelligence.
B. Microsoft’s strategic AI investments
Microsoft, once primarily known for its operating systems and office software, has transformed itself into a major player in the AI race through strategic investments, acquisitions, and partnerships. The company’s commitment to AI is evident across its product lines and research initiatives.
1. Azure AI and Cloud Services
Microsoft’s cloud platform, Azure, has become a cornerstone of its AI strategy:
- Azure Cognitive Services: This suite of AI services allows developers to easily integrate AI capabilities into their applications, including vision, speech, language, and decision-making functionalities.
- Azure Machine Learning: A cloud-based platform for building, training, and deploying machine learning models at scale.
- Azure AI Infrastructure: Microsoft has invested heavily in AI-optimized hardware and software infrastructure to support large-scale AI workloads.
2. OpenAI Partnership
One of Microsoft’s most significant AI moves was its partnership with OpenAI, a leading AI research organization:
- $1 Billion Investment: In 2019, Microsoft invested $1 billion in OpenAI, gaining exclusive access to GPT-3, one of the most advanced language models at the time.
- Expanded Partnership: In 2023, Microsoft further strengthened its ties with OpenAI through a multiyear, multibillion-dollar investment, reportedly worth $10 billion.
- Integration of OpenAI Technologies: Microsoft has begun integrating OpenAI’s technologies into its products, such as GitHub Copilot for code generation and the new AI-powered Bing search engine.
3. AI in Microsoft Products
Microsoft has been integrating AI across its product portfolio:
- Microsoft 365: AI-powered features in Office applications, such as Editor in Word, Designer in PowerPoint, and Insights in Excel, enhance productivity and creativity.
- Windows: AI capabilities in Windows 11, such as Windows Studio Effects for video calls and improved voice typing, demonstrate Microsoft’s commitment to AI-enhanced user experiences.
- Dynamics 365: Microsoft’s CRM and ERP solutions leverage AI for predictive analytics, customer insights, and process automation.
- Xbox and Gaming: AI is used to enhance gaming experiences, improve graphics, and create more realistic non-player characters (NPCs).
4. AI Research and Development
Microsoft Research has been at the forefront of AI innovation:
- Project Brainwave: An architecture for deep neural net processing that enables real-time AI calculations at scale.
- Conversational AI: Microsoft has made significant strides in natural language processing, leading to improvements in chatbots, virtual assistants, and language translation services.
- Computer Vision: Advancements in image and video recognition technologies have applications across various industries and products.
5. Acquisitions and Strategic Partnerships
Microsoft has bolstered its AI capabilities through strategic acquisitions and partnerships:
- LinkedIn Acquisition: The $26.2 billion acquisition of LinkedIn in 2016 provided Microsoft with vast amounts of professional data, enhancing its AI-powered recruitment and business networking services.
- Nuance Communications: In 2021, Microsoft acquired Nuance for $19.7 billion, strengthening its position in conversational AI and healthcare-specific AI applications.
- Collaboration with NVIDIA: Microsoft partnered with NVIDIA to build one of the world’s most powerful AI supercomputers to advance generative AI.
6. Ethical AI and Responsible Innovation
Microsoft has taken steps to address the ethical implications of AI:
- AI, Ethics, and Effects in Engineering and Research (AETHER) Committee: Established to ensure Microsoft’s AI technologies are developed and deployed responsibly.
- Responsible AI Standard: A framework for building AI systems with fairness, inclusiveness, reliability, safety, transparency, and privacy in mind.
7. AI for Good Initiatives
Microsoft has launched several programs aimed at using AI to address global challenges:
- AI for Earth: A $50 million program to support organizations using AI to solve environmental challenges.
- AI for Accessibility: An initiative to empower people with disabilities through AI-powered technologies.
- AI for Humanitarian Action: A program focused on using AI to support disaster response, protect children, promote human rights, and support refugees and displaced people.
8. Recent Developments and Future Focus
Microsoft continues to innovate and invest in AI:
- AI-powered Bing and Edge: The integration of ChatGPT-like capabilities into Bing search and Edge browser represents a significant push into AI-powered web search and browsing.
- Azure OpenAI Service: This offering allows businesses to access OpenAI’s advanced language models through Azure, with added security and compliance features.
- Copilot Integration: Microsoft is integrating AI-powered Copilot assistants across its product line, including Windows, Office, and GitHub, to enhance productivity and creativity.
Microsoft AI Initiative | Description | Impact |
---|---|---|
Azure AI | Cloud-based AI services and infrastructure | Enables businesses to leverage AI at scale |
OpenAI Partnership | Multibillion-dollar investment and collaboration | Access to cutting-edge AI models and research |
AI in Microsoft Products | Integration of AI across product portfolio | Enhanced user experiences and productivity |
Microsoft Research | In-house AI research and development | Advancements in various AI domains |
Strategic Acquisitions | LinkedIn, Nuance Communications | Expanded data access and domain-specific AI capabilities |
Ethical AI Initiatives | AETHER Committee, Responsible AI Standard | Promotion of responsible AI development and deployment |
AI for Good Programs | AI for Earth, Accessibility, Humanitarian Action | Application of AI to address global challenges |
AI-powered Bing and Edge | Integration of advanced language models | Transformation of web search and browsing experiences |
Microsoft’s strategic investments in AI, spanning cloud services, research, partnerships, and product integration, have positioned the company as a formidable competitor in the AI arms race. By leveraging its vast resources, extensive user base, and partnerships with leading AI research organizations, Microsoft is driving innovation across various AI domains and shaping the future of technology.
C. Meta’s AI research and development
Meta (formerly Facebook) has made significant strides in AI research and development, recognizing the technology’s potential to transform social media, virtual reality, and other areas of digital interaction. The company’s investments in AI span multiple domains and have led to several groundbreaking innovations.
1. Facebook AI Research (FAIR)
Established in 2013, FAIR is Meta’s dedicated AI research division:
- Long-term Research: FAIR focuses on advancing the state of the art in AI, with a particular emphasis on machine learning, computer vision, natural language processing, and robotics.
- Open Research Model: FAIR follows an open research model, publishing many of its findings and open-sourcing key technologies, contributing to the broader AI community.
- Global Presence: With labs in multiple countries, FAIR attracts top AI talent from around the world, fostering international collaboration and innovation.
2. PyTorch
One of Meta’s most significant contributions to the AI community is PyTorch:
- Open-source Framework: PyTorch is a machine learning framework that has gained widespread adoption in both academia and industry.
- Dynamic Computation Graphs: PyTorch’s dynamic computation graph approach offers flexibility and ease of use, making it popular for research and prototyping.
- Ecosystem Growth: Meta has invested in growing the PyTorch ecosystem, including tools for model deployment, optimization, and mobile development.
3. AI in Social Media and Content Moderation
Meta leverages AI extensively across its social media platforms:
- News Feed Personalization: AI algorithms power the personalized content recommendations on Facebook and Instagram.
- Content Moderation: AI systems help detect and remove harmful content, including hate speech, violence, and misinformation.
- Advertising: AI models optimize ad targeting and delivery, improving relevance for users and effectiveness for advertisers.
4. Computer Vision and Augmented Reality
Meta has made significant investments in computer vision and AR technologies:
- DeepFace: An advanced facial recognition system that approaches human-level accuracy.
- Spark AR: A platform for creating augmented reality experiences, powered by AI for features like body tracking and object recognition.
- AI-powered Image Understanding: Technologies for automatically describing images for visually impaired users and improving image search capabilities.
5. Natural Language Processing
Meta’s NLP research has led to several breakthroughs:
- RoBERTa: An optimized version of BERT (Bidirectional Encoder Representations from Transformers) that achieves state-of-the-art performance on various NLP tasks.
- Multilingual Translation: Development of advanced machine translation models capable of translating between multiple languages simultaneously.
- Conversational AI: Research into more natural and context-aware chatbots and virtual assistants.
6. AI for Virtual and Augmented Reality
As part of its metaverse vision, Meta is investing heavily in AI for VR and AR:
- Realistic Avatars: AI-powered technologies for creating and animating realistic digital avatars.
- Environmental Understanding: AI systems for mapping and understanding 3D spaces in real-time.
- Neural Interfaces: Research into brain-computer interfaces for more intuitive VR/AR interactions.
7. AI Hardware Development
Meta is developing custom hardware to support its AI ambitions:
- AI Research SuperCluster (RSC): One of the world’s fastest AI supercomputers, designed to train massive AI models.
- Custom AI Chips: Development of specialized AI processors for more efficient AI computations in data centers and edge devices.
8. Responsible AI and Ethics
Meta has established initiatives to address the ethical implications of AI:
- Responsible AI Framework: Guidelines and principles for developing AI systems that are fair, transparent, and accountable.
- AI Ethics Research: Funding and conducting research into the societal impacts of AI and potential mitigation strategies.
9. Recent Developments and Future Focus
Meta continues to push the boundaries of AI research and application:
- Large Language Models: Development of advanced language models like OPT (Open Pretrained Transformer) and LLaMA (Large Language Model Meta AI).
- AI for the Metaverse: Ongoing research into AI technologies that will power immersive virtual environments and experiences.
- AI for Climate Change: Initiatives using AI to address climate change, including the Climate Change Information Center and carbon footprint reduction efforts.
Meta AI Initiative | Description | Impact |
---|---|---|
FAIR | Dedicated AI research division | Advancements in ML, CV, NLP, and robotics |
PyTorch | Open-source ML framework | Widely adopted tool for AI development and research |
Content Moderation AI | AI systems for detecting harmful content | Improved safety and user experience on social platforms |
Computer Vision & AR | DeepFace, Spark AR | Enhanced facial recognition and AR experiences |
NLP Advancements | RoBERTa, multilingual translation | Improved language understanding and translation capabilities |
VR/AR AI | Avatar creation, environmental understanding | Foundation for metaverse technologies |
AI Research SuperCluster | High-performance AI computing infrastructure | Enables training of massive AI models |
Responsible AI Initiatives | Ethical guidelines and research | Promotion of fair and accountable AI development |
Meta’s multifaceted approach to AI research and development, combined with its vast user base and data resources, positions the company as a major player in the AI arms race. From fundamental research to practical applications in social media, virtual reality, and beyond, Meta’s AI initiatives are shaping the future of digital interaction and immersive technologies.
D. Amazon’s AI-powered innovations
Amazon, the e-commerce giant turned tech conglomerate, has become a formidable player in the AI arms race. The company’s AI initiatives span multiple domains, from enhancing its core retail business to powering cloud services and smart home devices. Amazon’s strategic investments in AI have
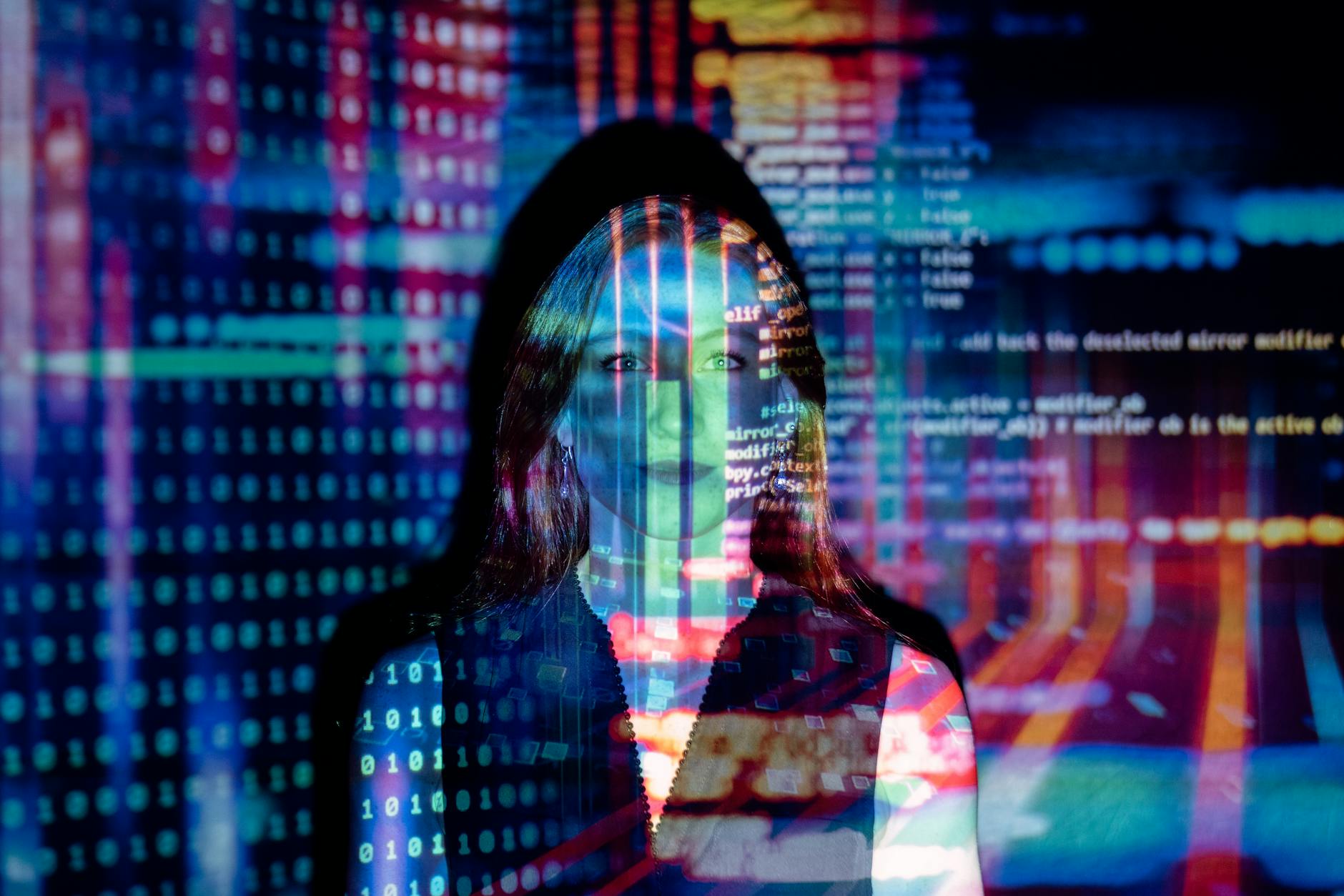
Key AI Technologies Driving Competition
As we delve into the heart of the AI arms race among big tech companies, it’s crucial to understand the key technologies that are fueling this fierce competition. These cutting-edge advancements are not only shaping the future of artificial intelligence but also determining which companies will lead the charge in this technological revolution.
A. Natural Language Processing Advancements
Natural Language Processing (NLP) has become one of the most hotly contested battlegrounds in the AI arms race. This technology, which enables machines to understand, interpret, and generate human language, has seen remarkable progress in recent years, driving innovation across various sectors.
1. Transformer Models and Their Impact
At the forefront of NLP advancements are transformer models, which have revolutionized the field since their introduction in 2017. These models, such as BERT, GPT, and T5, have demonstrated unprecedented capabilities in language understanding and generation.
- BERT (Bidirectional Encoder Representations from Transformers): Developed by Google, BERT has significantly improved search results and language understanding tasks.
- GPT (Generative Pre-trained Transformer): OpenAI’s GPT series, particularly GPT-3 and GPT-4, have showcased remarkable text generation abilities, leading to applications in content creation, coding assistance, and more.
- T5 (Text-to-Text Transfer Transformer): Google’s T5 model has shown impressive versatility across various NLP tasks, from translation to summarization.
The impact of these models on the AI landscape cannot be overstated. They have enabled more natural and context-aware language interactions, paving the way for more sophisticated chatbots, virtual assistants, and language translation services.
2. Multilingual and Cross-lingual Models
As the AI race extends globally, tech giants are focusing on developing multilingual and cross-lingual NLP models. These models can understand and generate text in multiple languages, breaking down language barriers and expanding the reach of AI-powered services.
- XLM-R (Cross-lingual Language Model – Roberta): Facebook’s XLM-R has shown remarkable performance across 100 languages, demonstrating the potential for truly global AI applications.
- M2M-100: Another Facebook innovation, this model can directly translate between any pair of 100 languages without relying on English as an intermediate step.
3. Zero-shot and Few-shot Learning
The ability of NLP models to perform tasks with minimal or no specific training data has become a key focus in the AI arms race. Zero-shot and few-shot learning capabilities are pushing the boundaries of what’s possible in language AI.
- GPT-3’s Few-shot Learning: OpenAI’s GPT-3 has demonstrated the ability to perform new tasks with just a few examples, revolutionizing the way we think about AI adaptability.
- CLIP (Contrastive Language-Image Pre-training): This OpenAI model can perform zero-shot image classification based on natural language descriptions, bridging the gap between vision and language AI.
4. Emotion and Sentiment Analysis
As AI systems become more sophisticated, the ability to understand and respond to human emotions has become a crucial area of development. Advanced sentiment analysis techniques are being integrated into various applications, from customer service to mental health support.
- IBM Watson Tone Analyzer: This tool can detect emotions and communication styles in written text, enabling more empathetic AI interactions.
- Google’s BERT for Sentiment Analysis: Fine-tuned BERT models have shown state-of-the-art performance in sentiment analysis tasks across various domains.
5. Conversational AI and Dialogue Systems
The development of more natural and context-aware conversational AI is a key battleground in the tech giants’ race for AI dominance. These systems are becoming increasingly sophisticated, able to maintain coherent dialogues over extended interactions.
- Google’s LaMDA (Language Model for Dialogue Applications): This conversational AI model has demonstrated the ability to engage in open-ended conversations on a wide range of topics.
- Microsoft’s DialoGPT: Built on the GPT-2 architecture, DialoGPT has shown impressive capabilities in generating human-like responses in multi-turn conversations.
The advancements in NLP are not just academic achievements; they’re driving real-world applications and shaping the future of human-computer interaction. From more accurate translation services to AI-powered content creation tools, these technologies are at the heart of many products and services offered by big tech companies.
B. Computer Vision Breakthroughs
Computer Vision, the field of AI that enables machines to derive meaningful information from visual inputs, has seen remarkable progress in recent years. This technology is crucial in various applications, from autonomous vehicles to facial recognition systems, making it a key battleground in the AI arms race.
1. Convolutional Neural Networks (CNNs) and Their Evolution
CNNs have been the backbone of computer vision for years, but recent advancements have pushed their capabilities to new heights.
- EfficientNet: Google’s EfficientNet models have achieved state-of-the-art accuracy on image classification tasks while being significantly more efficient than previous models.
- ResNet and Its Variants: Microsoft’s ResNet architecture, which introduced skip connections to allow for deeper networks, continues to be improved upon with variants like ResNeXt and ResNeSt.
2. Object Detection and Segmentation
The ability to accurately detect and segment objects in images and video streams is crucial for many applications, from autonomous driving to augmented reality.
- YOLO (You Only Look Once): This real-time object detection system, originally developed by Joseph Redmon and Ali Farhadi, has seen continuous improvements, with YOLOv5 and YOLOR pushing the boundaries of speed and accuracy.
- Mask R-CNN: Facebook AI Research’s Mask R-CNN has set new standards for instance segmentation, enabling pixel-level identification of objects.
Model | Task | Key Features |
---|---|---|
YOLO | Object Detection | Real-time processing, high accuracy |
Mask R-CNN | Instance Segmentation | Pixel-level object identification |
EfficientDet | Object Detection | Scalable architecture, high efficiency |
3. Generative Adversarial Networks (GANs) in Vision
GANs have revolutionized the field of image generation and manipulation, leading to both exciting applications and concerning ethical implications.
- StyleGAN: Developed by NVIDIA, StyleGAN and its successors have produced incredibly realistic synthetic images, pushing the boundaries of what’s possible in image generation.
- Pix2Pix and CycleGAN: These models, developed by researchers including those from Berkeley AI Research, have enabled impressive image-to-image translation tasks.
4. 3D Computer Vision
As AR and VR technologies advance, 3D computer vision has become increasingly important in the AI arms race.
- NeRF (Neural Radiance Fields): This technology, which allows for the creation of photorealistic 3D scenes from a set of 2D images, has seen rapid advancement with contributions from various tech giants.
- Facebook’s Habitat: This platform for embodied AI research is pushing the boundaries of 3D perception and navigation.
5. Video Understanding and Action Recognition
The ability to understand and analyze video content is becoming increasingly important in the age of streaming and user-generated content.
- SlowFast Networks: Developed by Facebook AI Research, these networks have achieved state-of-the-art performance in video action recognition.
- Google’s AVA Dataset and Models: The Atomic Visual Actions (AVA) dataset and associated models have advanced the field of dense action recognition in videos.
6. Cross-modal Vision and Language Models
The integration of vision and language understanding is a growing focus in the AI arms race, enabling more natural interactions between humans and AI systems.
- DALL-E and DALL-E 2: OpenAI’s models can generate images from textual descriptions, showcasing the potential of cross-modal AI.
- Microsoft’s VinVL: This Vision-Language Pre-training model has achieved state-of-the-art performance on various vision-language tasks.
7. Efficient and Mobile Computer Vision
As AI moves increasingly to edge devices, the development of efficient computer vision models has become a key area of competition.
- MobileNet: Google’s MobileNet series of models are designed for efficient inference on mobile and embedded devices.
- Apple’s Core ML: This framework allows for the deployment of lightweight, efficient machine learning models on Apple devices.
The advancements in computer vision are driving innovations across various industries. From enhancing smartphone cameras to enabling autonomous vehicles, these technologies are at the forefront of the AI revolution. Big tech companies are investing heavily in computer vision research and development, recognizing its potential to transform numerous aspects of our daily lives and business operations.
C. Machine Learning Algorithms
Machine Learning (ML) algorithms form the backbone of many AI applications, and their continuous improvement is a critical focus in the AI arms race. These algorithms enable systems to learn from data, recognize patterns, and make decisions with minimal human intervention.
1. Deep Learning and Neural Networks
Deep Learning, a subset of machine learning based on artificial neural networks, has been at the forefront of many recent AI breakthroughs.
- Transformer Architecture: Originally introduced for NLP tasks, transformers have found applications in various domains, including computer vision and time series analysis.
- Graph Neural Networks (GNNs): These networks, which can operate on graph-structured data, are being used in recommendation systems, social network analysis, and molecular property prediction.
2. Reinforcement Learning
Reinforcement Learning (RL) algorithms, which learn through interaction with an environment, have shown remarkable capabilities in complex decision-making tasks.
- DeepMind’s AlphaGo and AlphaFold: These systems have achieved superhuman performance in the game of Go and protein structure prediction, respectively, showcasing the power of advanced RL techniques.
- OpenAI’s Dota 2 AI: This system demonstrated the ability to compete at a professional level in the complex strategy game Dota 2.
3. Federated Learning
As privacy concerns grow, Federated Learning has emerged as a crucial technology allowing machine learning models to be trained on distributed datasets without centralizing the data.
- Google’s Federated Learning of Cohorts (FLoC): This technology aims to enable interest-based advertising without third-party cookies, balancing personalization and privacy.
- Apple’s on-device machine learning: Apple utilizes federated learning techniques to improve services like QuickType and Siri while keeping user data on their devices.
4. Meta-Learning and Few-Shot Learning
The ability to learn from limited data is becoming increasingly important in the AI arms race, leading to advancements in meta-learning and few-shot learning algorithms.
- Model-Agnostic Meta-Learning (MAML): This algorithm, developed by researchers including Chelsea Finn, enables models to quickly adapt to new tasks with minimal training data.
- Prototypical Networks: These networks have shown impressive performance in few-shot classification tasks across various domains.
5. Automated Machine Learning (AutoML)
AutoML technologies, which automate the process of selecting and optimizing machine learning models, are becoming increasingly sophisticated.
- Google’s Cloud AutoML: This suite of ML products allows developers with limited ML expertise to train high-quality models specific to their business needs.
- Microsoft’s Azure Automated Machine Learning: This service automates time-consuming tasks of ML model development, enabling data scientists to build models more efficiently.
6. Explainable AI (XAI)
As AI systems become more complex and are deployed in critical applications, the need for interpretable and explainable models has grown.
- LIME (Local Interpretable Model-agnostic Explanations): This technique provides local explanations for the predictions of any machine learning classifier.
- SHAP (SHapley Additive exPlanations): Based on game theory, SHAP assigns each feature an importance value for a particular prediction.
7. Quantum Machine Learning
Although still in its early stages, quantum machine learning is an area of intense research and competition among tech giants.
- Google’s Quantum Supremacy Experiment: While not directly related to machine learning, this experiment showcased the potential of quantum computing for solving certain problems exponentially faster than classical computers.
- IBM’s Qiskit Machine Learning: This open-source library allows researchers to experiment with quantum algorithms for machine learning tasks.
Here’s a comparison of some key machine learning paradigms:
Paradigm | Key Features | Example Applications |
---|---|---|
Deep Learning | Hierarchical feature learning, large data requirements | Image recognition, natural language processing |
Reinforcement Learning | Learning through interaction, suited for sequential decision-making | Game AI, robotics, autonomous systems |
Federated Learning | Privacy-preserving, distributed learning | Mobile keyboard prediction, healthcare |
Meta-Learning | Fast adaptation to new tasks, efficient use of data | Few-shot learning, continual learning |
The advancements in machine learning algorithms are driving innovations across various sectors, from healthcare to finance to entertainment. Big tech companies are investing heavily in research and development of novel ML techniques, recognizing their potential to create more intelligent and capable AI systems.
D. Robotics and Automation
Robotics and automation represent a critical frontier in the AI arms race, with potential applications ranging from manufacturing and logistics to healthcare and space exploration. The integration of advanced AI algorithms with physical systems is opening up new possibilities and challenges.
1. Autonomous Robots
The development of robots that can operate independently in complex, unstructured environments is a key focus area for many tech giants.
- Boston Dynamics (now owned by Hyundai): Their humanoid and quadruped robots, such as Atlas and Spot, showcase advanced locomotion and manipulation capabilities.
- Amazon’s warehouse robots: These autonomous mobile robots are revolutionizing warehouse operations and logistics.
2. Collaborative Robots (Cobots)
Cobots are designed to work alongside humans, combining the strengths of human workers with the precision and tirelessness of robots.
- Universal Robots: Their cobot arms are widely used in manufacturing and have set standards for human-robot collaboration.
- Google’s everyday robots: These robots are designed to operate in unstructured environments and assist with everyday tasks.
3. Soft Robotics
Soft robotics, which uses compliant materials mimicking biological systems, is an emerging field with promising applications in various domains.
- OctoBot: Developed by Harvard researchers, this soft, autonomous robot showcases the potential of soft robotics for delicate manipulation tasks.
- Soft Robotics Inc.: Their grippers, inspired by octopus tentacles, are used in food handling and other delicate operations.
4. Swarm Robotics
Swarm robotics involves coordinating large numbers of relatively simple robots to perform complex tasks collectively.
- Alphabet’s Project Wing: This drone delivery project utilizes swarm intelligence for efficient route planning and obstacle avoidance.
- Harvard’s Kilobot project: These small robots demonstrate how simple units can collaborate to form complex structures and behaviors.
5. Industrial Automation
Industrial automation is being transformed by AI, with smart factories and Industry 4.0 concepts driving innovation.
- Siemens’ MindSphere: This cloud-based IoT operating system connects products, plants, systems, and machines, enabling them to harness the wealth of data generated by the Internet of Things (IoT).
- ABB’s YuMi: This collaborative robot is designed for small parts assembly, working safely alongside humans in production environments.
6. Healthcare Robotics
The integration of AI and robotics in healthcare is opening up new possibilities for patient care, surgery, and rehabilitation.
- Intuitive Surgical’s da Vinci System: This robotic surgical system enhances the capabilities of surgeons, allowing for minimally invasive procedures.
- Cyberdyne’s HAL (Hybrid Assistive Limb): This exoskeleton suit aids mobility for patients with lower limb disabilities.
7. Autonomous Vehicles
While not traditionally considered “robots,” autonomous vehicles represent one of the most visible and impactful areas of AI-driven automation.
- Waymo (Google’s sister company): Their self-driving technology has logged millions of miles on public roads and is being deployed in ride-hailing services.
- Tesla’s Autopilot and Full Self-Driving (FSD): These systems are pushing the boundaries of what’s possible with consumer-grade autonomous driving technology.
8. Robotic Process Automation (RPA)
RPA uses software robots or “bots” to automate repetitive, rule-based digital tasks.
- UiPath: Their RPA platform allows organizations to automate various business processes, from data entry to customer service.
- Blue Prism: This company pioneered the term “Robotic Process Automation” and provides enterprise-grade automation solutions.
Here’s a comparison of different robotics and automation paradigms:
Paradigm | Key Features | Example Applications |
---|---|---|
Autonomous Robots | Independent operation, complex decision-making | Warehouse automation, exploration |
Collaborative Robots | Safe human-robot interaction, flexibility | Manufacturing, assembly lines |
Soft Robotics | Compliance, adaptability to unstructured environments | Delicate manipulation, medical applications |
Swarm Robotics | Collective behavior, scalability | Search and rescue, environmental monitoring |
Industrial Automation | High precision, efficiency, data-driven optimization | Smart factories, process industries |
The field of robotics and automation is rapidly evolving, driven by advancements in AI, materials science, and mechatronics. Big tech companies are investing heavily in this area, recogn
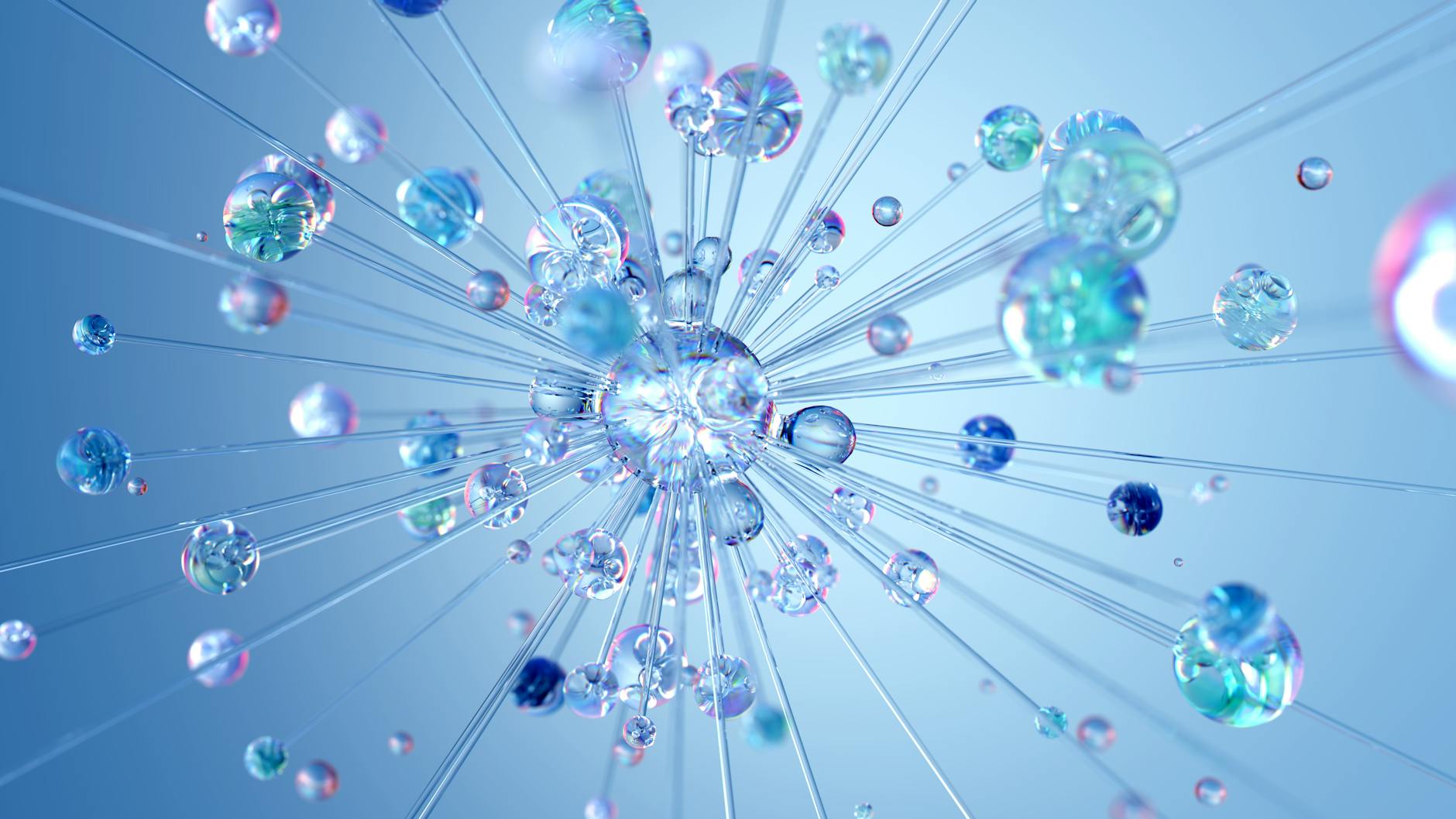
The Race for AI Talent
As we delve into the fierce competition for AI talent among big tech companies, it’s crucial to understand that the success of any AI initiative largely depends on the quality and expertise of the human minds behind it. The race for AI talent has become one of the most critical aspects of the AI arms race, with companies employing various strategies to attract, retain, and nurture the best minds in the field.
A. Competitive recruitment strategies
In the ever-evolving landscape of artificial intelligence, the demand for top-tier AI talent far outweighs the supply. This scarcity has led to an unprecedented war for talent, with big tech companies pulling out all the stops to secure the brightest minds in the field. Let’s explore some of the most effective and innovative recruitment strategies employed by industry leaders.
1. Competitive compensation packages
At the forefront of recruitment strategies is the offering of highly competitive compensation packages. These packages often include:
- Base salaries that significantly exceed industry averages
- Performance-based bonuses tied to project success and company growth
- Stock options and equity grants to align employee interests with company success
- Sign-on bonuses to incentivize immediate acceptance of job offers
For example, according to a recent industry report, AI specialists at top tech companies can command salaries ranging from $300,000 to over $1 million per year, depending on their experience and expertise. This level of compensation reflects the critical importance of AI talent in driving innovation and maintaining a competitive edge.
2. Cutting-edge research opportunities
Many AI professionals are driven by the desire to push the boundaries of what’s possible in the field. To attract these individuals, companies are offering:
- Access to state-of-the-art research facilities and computing resources
- Opportunities to work on groundbreaking projects with real-world impact
- Collaboration with other leading experts in the field
- Freedom to publish research findings and contribute to the broader AI community
Google’s AI research division, Google Brain, is a prime example of this strategy in action. The company has created an environment where researchers can work on cutting-edge problems while also maintaining academic connections through publications and conference presentations.
3. Work-life balance and flexible working arrangements
Recognizing the importance of work-life balance, especially for highly sought-after professionals, companies are offering:
- Flexible working hours and remote work options
- Extended paid time off and sabbatical programs
- On-site amenities such as gyms, meditation rooms, and gourmet cafeterias
- Family-friendly policies, including extended parental leave and childcare support
These benefits not only attract talent but also contribute to long-term retention by fostering a positive work environment and supporting employees’ overall well-being.
4. Career development and continuous learning
To appeal to AI professionals’ desire for growth and advancement, companies are implementing:
- Structured career progression paths within AI-related roles
- Mentorship programs pairing junior talent with experienced AI leaders
- Regular training and development opportunities, including workshops and conferences
- Internal hackathons and innovation challenges to spark creativity
Microsoft’s AI Residency Program is an excellent example of this approach. The program offers recent graduates and professionals from other fields the opportunity to work on real-world AI projects while receiving mentorship and training from industry experts.
5. Employer branding and thought leadership
Companies are investing heavily in building their reputation as AI leaders through:
- Hosting and sponsoring major AI conferences and events
- Publishing whitepapers and research articles on AI advancements
- Engaging in public discussions about AI ethics and responsible development
- Showcasing AI projects and their impact on society
For instance, OpenAI, while not a traditional “big tech” company, has built a strong reputation in the AI community through its groundbreaking research and commitment to developing safe and beneficial AI.
6. Global talent acquisition
Recognizing that AI talent is not confined to any single geographic location, companies are:
- Establishing AI research centers in multiple countries
- Offering relocation packages and visa sponsorship for international talent
- Implementing remote-first hiring practices to tap into global talent pools
- Partnering with universities worldwide to identify and nurture emerging talent
Google’s decision to open AI research centers in locations such as Accra, Ghana, and Tokyo, Japan, demonstrates this global approach to talent acquisition.
7. Referral programs and network leveraging
Companies are tapping into their existing talent pool to attract new AI professionals by:
- Offering substantial referral bonuses for successful hires
- Encouraging employees to act as brand ambassadors at industry events
- Leveraging alumni networks of top AI researchers and engineers
- Creating online communities and forums for AI professionals to connect and share ideas
8. Tailored recruitment processes
To streamline the hiring of AI talent, companies are implementing:
- AI-powered resume screening and candidate matching tools
- Technical assessments and coding challenges specific to AI roles
- Accelerated interview processes for high-priority candidates
- Personalized outreach and recruitment strategies for top-tier talent
9. Retention strategies
While attracting talent is crucial, retaining it is equally important. Companies are focusing on:
- Regular salary reviews and market adjustments
- Creating a culture of innovation and continuous learning
- Offering opportunities for internal mobility within AI-related projects
- Recognizing and rewarding contributions to the field through awards and promotions
10. Diversity and inclusion initiatives
Recognizing the importance of diverse perspectives in AI development, companies are:
- Implementing targeted recruitment programs for underrepresented groups in tech
- Partnering with organizations that promote diversity in AI and STEM fields
- Offering scholarships and internships to support diverse talent pipelines
- Creating inclusive work environments that celebrate different backgrounds and ideas
Here’s a comparison of some key recruitment strategies employed by major tech companies:
Company | Key Recruitment Strategies |
---|---|
Research opportunities, Global AI centers, Work-life balance | |
Microsoft | AI Residency Program, Career development, Competitive compensation |
Amazon | Internal AI training programs, Remote work options, Innovation challenges |
Research publications, Hackathons, Collaboration with academia | |
Apple | Cutting-edge projects, Privacy focus, Competitive benefits |
The race for AI talent is intensifying as companies recognize the critical role that skilled professionals play in advancing AI technologies. By employing a combination of these strategies, big tech companies are not only attracting top talent but also creating environments where AI professionals can thrive and innovate.
B. Acquisitions of AI startups
As the AI arms race heats up, big tech companies are not just relying on traditional recruitment methods to secure top talent. They’re also turning to strategic acquisitions of AI startups as a way to rapidly expand their AI capabilities and bring in new teams of skilled professionals. This approach has become a crucial component of the overall talent acquisition strategy for many tech giants.
1. The strategic value of AI startup acquisitions
Acquiring AI startups offers several advantages for big tech companies:
- Immediate access to specialized AI talent and expertise
- Acquisition of proprietary AI technologies and intellectual property
- Expansion into new AI-related markets or applications
- Elimination of potential future competitors
- Acceleration of internal AI development timelines
2. Notable AI startup acquisitions by big tech
Let’s examine some of the most significant AI startup acquisitions made by major tech companies in recent years:
Google (Alphabet)
- DeepMind (2014): $500 million
- Purpose: Advanced AI research and development
- Impact: Brought in a team of world-class AI researchers and led to breakthroughs like AlphaGo
- Kaggle (2017): Undisclosed amount
- Purpose: Data science and machine learning community
- Impact: Enhanced Google’s AI ecosystem and talent pool
Microsoft
- SwiftKey (2016): $250 million
- Purpose: AI-powered keyboard technology
- Impact: Improved Microsoft’s mobile typing experience and natural language processing capabilities
- Nuance Communications (2021): $19.7 billion
- Purpose: Speech recognition and conversational AI
- Impact: Bolstered Microsoft’s position in healthcare AI and voice recognition technology
Facebook (Meta)
- Ozlo (2017): Undisclosed amount
- Purpose: Conversational AI and virtual assistants
- Impact: Enhanced Facebook’s natural language processing capabilities
- Bloomsbury AI (2018): $23-30 million
- Purpose: Natural language processing and question-answering systems
- Impact: Improved Facebook’s ability to combat fake news and misinformation
Apple
- Turi (2016): $200 million
- Purpose: Machine learning platform
- Impact: Strengthened Apple’s AI and machine learning capabilities across its product lineup
- Xnor.ai (2020): $200 million
- Purpose: Edge-based AI tools
- Impact: Enhanced Apple’s ability to run AI algorithms efficiently on devices with limited resources
Amazon
- Kiva Systems (2012): $775 million
- Purpose: Robotics and automation for warehouses
- Impact: Revolutionized Amazon’s warehouse operations and logistics
- Ring (2018): $1 billion
- Purpose: Smart home security devices with AI capabilities
- Impact: Expanded Amazon’s presence in the smart home market and enhanced its AI-powered security offerings
3. Integration challenges and strategies
While acquiring AI startups can provide immediate access to talent and technology, integrating these companies into larger organizations presents its own set of challenges:
- Cultural differences between startup and corporate environments
- Aligning the acquired team’s goals with the parent company’s objectives
- Retaining key talent post-acquisition
- Integrating different technology stacks and development processes
To address these challenges, big tech companies often employ the following strategies:
- Maintaining the startup’s autonomy and brand identity (e.g., Google’s approach with DeepMind)
- Offering retention bonuses and long-term incentives to key employees
- Providing resources and support while minimizing bureaucratic interference
- Creating cross-functional teams to facilitate knowledge transfer and collaboration
4. The impact on the AI startup ecosystem
The trend of big tech companies acquiring AI startups has had significant effects on the broader AI ecosystem:
- Increased investment in AI startups, as founders and investors see potential for lucrative exits
- Acceleration of AI innovation as startups compete to develop unique technologies
- Concentration of AI talent and intellectual property within large tech companies
- Potential reduction in long-term competition as promising startups are absorbed by industry giants
5. Regulatory considerations
As big tech companies continue to acquire AI startups, regulators are taking notice:
- Antitrust concerns over market concentration in the AI sector
- Scrutiny of data privacy implications when large companies acquire startups with access to user data
- Calls for increased oversight of AI-related mergers and acquisitions
6. Future trends in AI startup acquisitions
Looking ahead, we can expect to see:
- Increased competition for AI startups from non-tech companies seeking to bolster their AI capabilities
- More targeted acquisitions focusing on specific AI domains (e.g., healthcare AI, autonomous vehicles)
- Greater emphasis on acqui-hires, where the primary goal is to obtain talent rather than technology
- Potential emergence of new players in the AI field through strategic acquisitions
7. Case study: DeepMind acquisition by Google
To illustrate the impact of AI startup acquisitions, let’s take a closer look at Google’s acquisition of DeepMind:
- Acquisition details:
- Date: January 2014
- Price: $500 million
- Purpose: To bolster Google’s AI research capabilities
- Key outcomes:
- Development of AlphaGo, which defeated the world champion in the game of Go
- Advancements in protein folding prediction with AlphaFold
- Application of AI to improve Google’s products, including energy efficiency in data centers
- Challenges:
- Maintaining DeepMind’s independence while aligning with Google’s goals
- Addressing ethical concerns and public scrutiny of AI development
- Lessons learned:
- The importance of allowing acquired companies to maintain their culture and research focus
- The potential for breakthrough innovations when combining startup agility with big tech resources
8. Comparison of acquisition strategies
Here’s a comparison of AI startup acquisition strategies among big tech companies:
Company | Acquisition Focus | Integration Approach | Notable Acquisitions |
---|---|---|---|
Research-oriented | Semi-autonomous | DeepMind, Kaggle | |
Microsoft | Product-focused | Full integration | SwiftKey, Nuance |
Enhancing existing products | Mixed approach | Ozlo, Bloomsbury AI | |
Apple | Hardware/software synergy | Tight integration | Turi, Xnor.ai |
Amazon | Practical AI applications | Varies by acquisition | Kiva Systems, Ring |
The acquisition of AI startups has become a critical strategy in the race for AI talent and technology. By carefully selecting and integrating these companies, big tech firms are not only expanding their AI capabilities but also shaping the future of the AI industry. As this trend continues, we can expect to see further consolidation in the AI sector, with potential implications for innovation, competition, and regulation in the years to come.
C. Partnerships with academic institutions
As the competition for AI talent intensifies, big tech companies are increasingly turning to academic partnerships as a strategic approach to nurture and attract top AI talent. These collaborations between industry and academia serve multiple purposes, from advancing cutting-edge research to creating pipelines for future talent acquisition. Let’s explore the various aspects of these partnerships and their impact on the AI talent landscape.
1. Types of academic partnerships
Big tech companies engage in various forms of collaboration with universities and research institutions:
Research collaborations
- Joint research projects between company scientists and academic researchers
- Funding for specific research initiatives or labs within universities
- Access to company data and resources for academic research purposes
Educational programs
- Development of AI-focused curricula and courses
- Sponsorship of AI-related degree programs or specializations
- Provision of guest lectures and practical workshops by industry experts
Internship and fellowship programs
- Offering internships to promising students in AI-related fields
- Establishing fellowship programs for graduate students and postdoctoral researchers
- Creating opportunities for faculty sabbaticals within company research labs
Hackathons and competitions
- Organizing AI-focused hackathons and coding competitions for students
- Sponsoring academic AI challenges with real-world applications
Infrastructure support
- Providing cloud computing resources and AI tools to universities
- Donating hardware (e.g., GPUs) to support AI research and education
2. Benefits of academic partnerships
These collaborations offer numerous advantages for both tech companies and academic institutions:
For tech companies:
- Early access to cutting-edge research and emerging AI technologies
- Opportunity to shape the skills and knowledge of future AI professionals
- Enhanced reputation within the academic community
- Pipeline for identifying and recruiting top AI talent
- Fresh perspectives and innovative ideas from academic researchers
For academic institutions:
- Access to industry-scale data and computing resources
- Funding for research projects and infrastructure
- Real-world application opportunities for theoretical research
- Enhanced employability for students in AI-related programs
- Exposure to industry best practices and current market needs
3. Notable academic partnerships in AI
Let’s examine some prominent partnerships between big tech companies and academic institutions:
Google AI Research
- Partnership with Stanford University’s Human-Centered AI Institute
- Collaboration with MIT on the MIT-Google Brain project
- Support for the Berkeley Artificial Intelligence Research (BAIR) Lab
Microsoft Research
- Microsoft Research-Cambridge University Machine Learning Initiative
- Partnership with Carnegie Mellon University on conversational AI
- Collaboration with the University of Washington on quantum computing and AI
Facebook AI Research (FAIR)
- Partnerships with NYU, UC Berkeley, and Georgia Tech on various AI research projects
- Collaboration with the Montreal Institute for Learning Algorithms (MILA)
IBM Research
- IBM-MIT Laboratory for Brain-inspired Multimedia Machine Comprehension
- Collaboration with the University of Illinois Urbana-Champaign on AI and quantum computing
Amazon Web Services (AWS)
- AWS Machine Learning Research Awards program supporting various universities
- Partnership with Carnegie Mellon University on voice technology research
4. Case study: Google Brain Residency Program
To illustrate the impact of academic partnerships, let’s take a closer look at the Google Brain Residency Program:
- Program overview:
- 12-month research training program for recent graduates and professionals
- Participants work alongside Google researchers on real-world machine learning problems
- Opportunity to publish research and attend major AI conferences
- Key outcomes:
- Development of cutting-edge AI technologies and research papers
- Creation of a pipeline for identifying and recruiting top AI talent
- Fostering a collaborative ecosystem between industry and academia
- Success metrics:
- Number of research publications resulting from the program
- Percentage of residents who join Google full-time after the program
- Impact on Google’s AI products and services
5. Challenges in academic partnerships
While these collaborations offer significant benefits, they also come with challenges:
- Balancing academic freedom with company objectives
- Addressing potential conflicts of interest in research
- Managing intellectual property rights and publication restrictions
- Ensuring equitable access to partnership opportunities across institutions
- Maintaining long-term commitment and consistent funding
6. Ethical considerations
As academic partnerships in AI become more prevalent, several ethical considerations arise:
- Ensuring transparency in research funding and potential biases
- Addressing concerns about the commercialization of academic research
- Balancing the pursuit of AI advancements with potential societal impacts
- Maintaining academic integrity and independence in collaborative projects
7. Future trends in academic partnerships
Looking ahead, we can expect to see:
- Increased focus on interdisciplinary AI research collaborations
- Greater emphasis on AI ethics and responsible AI development in partnerships
- Expansion of online and remote collaboration models for global partnerships
- Development of more structured talent pipelines from academia to
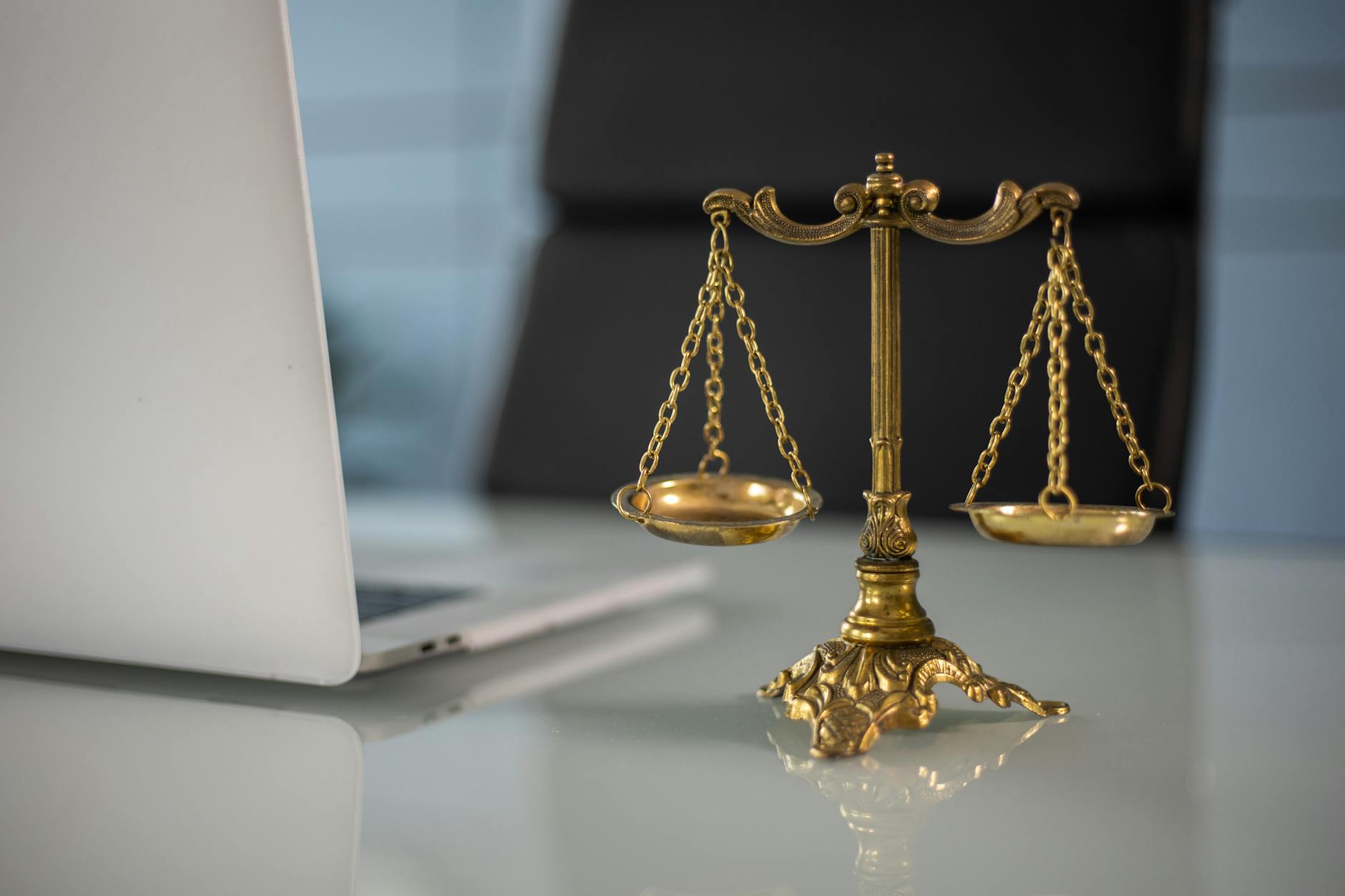
Ethical Concerns and Regulatory Challenges
As the AI arms race among big tech companies intensifies, it brings forth a myriad of ethical concerns and regulatory challenges that cannot be ignored. These issues are not only crucial for the companies involved but also for society as a whole. In this section, we’ll delve deep into the ethical considerations and regulatory hurdles that accompany the rapid advancement of AI technologies.
A. Bias and fairness in AI systems
One of the most pressing ethical concerns in the AI arms race is the issue of bias and fairness in AI systems. As companies rush to develop and deploy AI technologies, there’s a significant risk of perpetuating and even amplifying existing societal biases.
Understanding AI bias
AI bias refers to the systematic and repeatable errors in AI systems that create unfair outcomes. These biases can stem from various sources:
- Data bias: When the training data used to develop AI models is not representative of the entire population or contains historical biases.
- Algorithmic bias: When the algorithms themselves are designed in a way that favors certain groups over others.
- Interaction bias: When the way users interact with AI systems reinforces existing biases.
Examples of AI bias in real-world applications
To better understand the impact of AI bias, let’s look at some notable examples:
- Facial recognition: Studies have shown that many facial recognition systems perform poorly on people of color and women, leading to higher rates of misidentification.
- Hiring algorithms: AI-powered recruitment tools have been found to discriminate against certain groups, such as women or older applicants.
- Criminal justice: Risk assessment algorithms used in the criminal justice system have been criticized for being biased against minority groups.
Efforts to address AI bias
Big tech companies are increasingly aware of the need to address bias in their AI systems. Some initiatives include:
- Diverse teams: Ensuring that AI development teams are diverse and inclusive to bring different perspectives to the table.
- Bias testing: Implementing rigorous testing procedures to identify and mitigate bias in AI models before deployment.
- Transparency: Providing more information about how AI systems make decisions and allowing for external audits.
The importance of fairness in AI
Fairness in AI is not just an ethical imperative; it’s also crucial for building trust in these technologies. As AI becomes more prevalent in our daily lives, ensuring that these systems treat all individuals fairly is essential for their widespread adoption and acceptance.
Aspect of Fairness | Description | Importance |
---|---|---|
Equal treatment | AI systems should treat all individuals equally regardless of their race, gender, age, or other protected characteristics. | Prevents discrimination and promotes social equity |
Equal opportunity | AI should provide equal opportunities for all users, without favoring certain groups. | Ensures a level playing field and promotes meritocracy |
Equal outcome | The results produced by AI systems should be equitable across different demographic groups. | Helps to prevent the reinforcement of existing societal inequalities |
Transparency | The decision-making process of AI systems should be transparent and explainable. | Builds trust and allows for scrutiny of potential biases |
Challenges in achieving fairness
Despite the importance of fairness, achieving it in AI systems is not straightforward. Some challenges include:
- Defining fairness: There are multiple definitions of fairness, and they can sometimes be in conflict with each other.
- Trade-offs: Sometimes, improving fairness in one aspect can lead to decreased performance or fairness in another aspect.
- Contextual nature: What is considered fair can vary depending on the specific context and application of the AI system.
As the AI arms race continues, addressing bias and ensuring fairness in AI systems will remain a critical challenge for big tech companies. It requires ongoing vigilance, research, and collaboration between technologists, ethicists, and policymakers.
B. Privacy and data protection issues
As AI technologies become more sophisticated and pervasive, privacy and data protection have emerged as major concerns in the AI arms race. Big tech companies are constantly collecting and analyzing vast amounts of data to train their AI models and improve their services. However, this data-hungry approach raises significant privacy issues.
The data-AI nexus
AI systems, particularly machine learning models, require enormous amounts of data to function effectively. This creates a symbiotic relationship between data collection and AI development:
- More data leads to better AI performance
- Better AI performance leads to more user engagement
- More user engagement leads to more data collection
This cycle has led to an unprecedented accumulation of personal data by big tech companies.
Privacy concerns in AI
The extensive data collection and processing required for AI development raise several privacy concerns:
- Data collection: The sheer volume and variety of data collected can be intrusive and may exceed what users expect or are comfortable with.
- Data storage: Storing large amounts of personal data creates potential security risks if the data is breached.
- Data analysis: AI systems can derive sensitive insights from seemingly innocuous data, potentially revealing more about individuals than they intended to share.
- Data sharing: The sharing of data between companies or with third parties can lead to unexpected uses of personal information.
Specific privacy challenges in AI
Let’s delve deeper into some specific privacy challenges that arise in the context of AI:
- Re-identification: AI techniques can sometimes re-identify individuals from anonymized datasets, undermining privacy protections.
- Inference attacks: AI models can infer sensitive information about individuals, even if that information wasn’t explicitly provided.
- Model inversion: In some cases, it’s possible to extract training data from AI models, potentially exposing private information.
- Differential privacy: Ensuring that AI models don’t reveal information about specific individuals in their training data is a complex challenge.
Data protection regulations
In response to these privacy concerns, various data protection regulations have been implemented around the world. The most notable of these is the European Union’s General Data Protection Regulation (GDPR), which has set a new standard for data protection globally.
Key aspects of GDPR that impact AI development include:
- Consent: Users must give explicit consent for their data to be collected and processed.
- Purpose limitation: Data can only be used for the specific purposes for which it was collected.
- Data minimization: Only the minimum amount of data necessary should be collected and processed.
- Right to explanation: Users have the right to receive explanations for decisions made by automated systems.
- Right to be forgotten: Users can request that their personal data be erased.
Challenges in complying with data protection regulations
While data protection regulations are crucial for safeguarding privacy, they pose several challenges for companies engaged in the AI arms race:
- Balancing innovation and compliance: Companies must find ways to innovate with AI while staying within the bounds of data protection laws.
- Cross-border data flows: Different countries have different data protection laws, making it challenging to develop and deploy AI systems globally.
- Explainability: The “black box” nature of some AI models makes it difficult to provide the explanations required by regulations like GDPR.
- Data minimization: The data-hungry nature of AI is at odds with the principle of data minimization.
Privacy-enhancing technologies
To address these challenges, big tech companies are investing in privacy-enhancing technologies:
- Federated learning: This allows AI models to be trained on decentralized data, reducing the need to collect and store personal data centrally.
- Homomorphic encryption: This enables computations to be performed on encrypted data, protecting privacy even during processing.
- Differential privacy: This technique adds noise to datasets or model outputs to protect individual privacy while still allowing useful insights to be derived.
The future of privacy in AI
As the AI arms race continues, privacy and data protection will remain critical issues. Companies will need to:
- Invest in privacy-preserving AI techniques
- Develop robust data governance frameworks
- Engage with regulators and policymakers to shape responsible AI practices
- Educate users about how their data is used in AI systems
By addressing these privacy and data protection issues head-on, big tech companies can build trust with users and ensure the sustainable development of AI technologies.
C. Potential job displacement
As AI technologies continue to advance at a rapid pace, one of the most significant ethical concerns is the potential for widespread job displacement. The AI arms race among big tech companies is driving innovations that could automate many tasks currently performed by humans, leading to substantial changes in the job market.
Understanding AI-driven job displacement
AI-driven job displacement refers to the phenomenon where AI technologies and automation replace human workers in various industries and job roles. This displacement can take several forms:
- Full automation: Some jobs may be entirely automated, eliminating the need for human workers.
- Partial automation: AI may take over certain tasks within a job, reducing the number of human workers needed.
- Job transformation: Existing roles may evolve to incorporate AI, requiring new skills from workers.
Industries at risk
While AI has the potential to impact jobs across all sectors, some industries are particularly vulnerable to AI-driven displacement:
- Manufacturing: Robotics and AI-powered systems are increasingly capable of performing complex manufacturing tasks.
- Transportation: Self-driving vehicles could displace millions of drivers in the coming decades.
- Customer service: AI-powered chatbots and virtual assistants are taking over many customer service roles.
- Finance: Algorithmic trading and AI-powered financial analysis are reducing the need for human traders and analysts.
- Healthcare: AI is being used for diagnostics, drug discovery, and even some surgical procedures, potentially impacting healthcare jobs.
The scale of potential job displacement
Estimating the exact scale of AI-driven job displacement is challenging, as it depends on various factors including the pace of technological advancement, adoption rates, and economic conditions. However, several studies have attempted to quantify the potential impact:
Study | Estimated Impact | Timeframe |
---|---|---|
McKinsey Global Institute | Up to 800 million jobs displaced globally | By 2030 |
World Economic Forum | 75 million jobs displaced, 133 million new jobs created | By 2022 |
PwC | 30% of jobs at high risk of automation | By mid-2030s |
It’s important to note that while these studies predict significant job displacement, they also forecast the creation of new jobs related to AI and other emerging technologies.
Ethical implications of job displacement
The potential for widespread job displacement raises several ethical concerns:
- Economic inequality: Job losses due to AI could exacerbate existing economic inequalities if displaced workers struggle to find new employment.
- Social disruption: Mass unemployment could lead to social unrest and political instability.
- Mental health: Job loss can have severe psychological impacts on individuals and communities.
- Skill obsolescence: Rapid technological change may render many workers’ skills obsolete, requiring significant retraining efforts.
Strategies for mitigating job displacement
To address the ethical challenges posed by AI-driven job displacement, various strategies have been proposed:
- Education and retraining: Investing in education and retraining programs to help workers adapt to the changing job market.
- Universal Basic Income (UBI): Providing a basic income to all citizens to cushion the impact of job losses.
- Job guarantees: Government programs to ensure employment for those displaced by AI.
- Shorter work weeks: Redistributing available work by reducing the standard work week.
- AI-human collaboration: Focusing on developing AI systems that augment human capabilities rather than replace them entirely.
The role of big tech companies
As the primary drivers of AI innovation, big tech companies have a significant responsibility in addressing the potential for job displacement. Some actions they can take include:
- Investing in workforce development: Funding education and retraining programs for workers at risk of displacement.
- Responsible AI development: Prioritizing the development of AI systems that complement human workers rather than replace them.
- Job creation: Actively creating new roles within their organizations that leverage AI technologies.
- Transparency: Being open about the potential impacts of their AI technologies on employment.
- Collaboration with governments: Working with policymakers to develop strategies for managing the transition to an AI-driven economy.
The future of work in an AI-driven world
While the potential for job displacement is a serious concern, it’s important to recognize that AI also has the potential to create new jobs and industries. The key challenge will be managing the transition to ensure that the benefits of AI are broadly shared across society.
Some potential positive outcomes of AI in the workplace include:
- Increased productivity: AI can take over repetitive tasks, allowing humans to focus on more creative and strategic work.
- New job categories: AI will likely create entirely new job categories that we can’t yet envision.
- Improved working conditions: AI can help reduce workplace accidents and improve ergonomics.
- Enhanced human capabilities: AI tools can augment human intelligence, leading to better decision-making and problem-solving.
As the AI arms race continues, it’s crucial that big tech companies, policymakers, and society at large work together to harness the benefits of AI while mitigating its potential negative impacts on employment. This will require ongoing dialogue, adaptive policies, and a commitment to ethical AI development.
D. Regulatory frameworks and compliance
As AI technologies continue to advance and their impact on society grows, the need for comprehensive regulatory frameworks becomes increasingly apparent. The AI arms race among big tech companies has outpaced existing regulations, leading to a complex landscape of emerging policies and compliance challenges.
The current state of AI regulation
AI regulation is still in its infancy, with most countries and regions still in the process of developing comprehensive frameworks. However, some notable initiatives have emerged:
- European Union’s AI Act: A proposed regulation that aims to create a unified approach to AI governance across the EU.
- China’s AI Governance Principles: Guidelines for the ethical development and use of AI in China.
- U.S. AI Bill of Rights: A non-binding set of principles for the development and use of AI systems in the United States.
Key areas of AI regulation
AI regulations typically focus on several key areas:
- Transparency and explainability: Ensuring that AI systems’ decision-making processes are understandable and can be explained to affected individuals.
- Accountability: Establishing clear lines of responsibility for the outcomes of AI systems.
- Fairness and non-discrimination: Preventing AI systems from perpetuating or amplifying biases.
- Privacy and data protection: Safeguarding personal data used in AI systems.
- Safety and security: Ensuring AI systems are reliable and protected against malicious use.
Challenges in AI regulation
Regulating AI presents several unique challenges:
- Rapid technological advancement: AI technologies evolve faster than traditional regulatory processes can keep up.
- Complexity: The technical complexity of AI systems makes it difficult for regulators to fully understand and govern them.
- Global nature: AI development and deployment often cross national borders, requiring international cooperation.
- Balancing innovation and protection: Regulations must protect against potential harms without stifling innovation.
Compliance challenges for big tech companies
As regulatory frameworks for AI emerge, big tech companies face several compliance challenges:
- Varying global standards: Companies must navigate different regulatory requirements across jurisdictions.
- Implementing explainable AI: Making complex AI systems transparent and explainable can be technically challenging.
- Data governance: Ensuring compliance with data protection regulations while still collecting enough data to train effective AI models.
- Ethical AI development: Integrating ethical considerations into the AI development process from the outset.
- Continuous monitoring: AI systems can evolve over time, requiring ongoing monitoring for compliance.
Strategies for ensuring compliance
To navigate the complex regulatory landscape, big tech companies are adopting various strategies:
- Proactive engagement: Actively participating in policy discussions and helping shape AI regulations.
- Ethical AI frameworks: Developing internal guidelines and principles for ethical AI development.
- Cross-functional teams: Creating teams that combine technical, legal, and ethical expertise to address compliance issues.
- AI auditing: Implementing regular audits of AI systems to ensure ongoing compliance.
- Transparency initiatives: Providing more information about AI systems to users and regulators.
The role of self-regulation
Given the rapid pace of AI development, some argue that self-regulation by the tech industry is necessary to complement government regulations. Self-regulation efforts include:
- Industry standards: Developing voluntary standards for AI development and deployment.
- Ethics boards: Establishing external advisory boards to guide ethical AI development.
- Collaborative initiatives: Forming industry-wide collaborations to address common ethical and regulatory challenges.
Case study: The EU’s AI Act
To better understand the regulatory landscape, let’s examine the European Union’s proposed AI Act:
Aspect | Description |
---|---|
Risk-based approach | Categorizes AI systems based on their potential risk, with stricter requirements for high-risk applications. |
Prohibited AI practices | Bans certain AI applications, such as social scoring systems and real-time biometric identification in public spaces. |
Requirements for high-risk AI | Mandates risk assessments, human oversight, and transparency for high-risk AI systems. |
Penalties for non-compliance | Proposes fines of up to €30 million or 6% of global turnover for serious violations. |
AI Board | Establishes a European Artificial Intelligence Board to facilitate implementation and develop standards. |
The EU’s AI Act, if adopted, would set a global benchmark for AI regulation and likely influence regulatory approaches in other jurisdictions.
The future of AI regulation
As the AI arms race continues, we can expect to see:
- More comprehensive AI-specific regulations: Countries and regions will develop more detailed AI governance frameworks.
- Increased focus on AI ethics: Ethical considerations will become more central to AI regulation.
- International cooperation: Efforts to develop global standards for AI governance will intensify.
- Adaptive regulation: Regulatory approaches that can evolve alongside AI technologies will emerge.
- Sector-specific regulations: Different industries
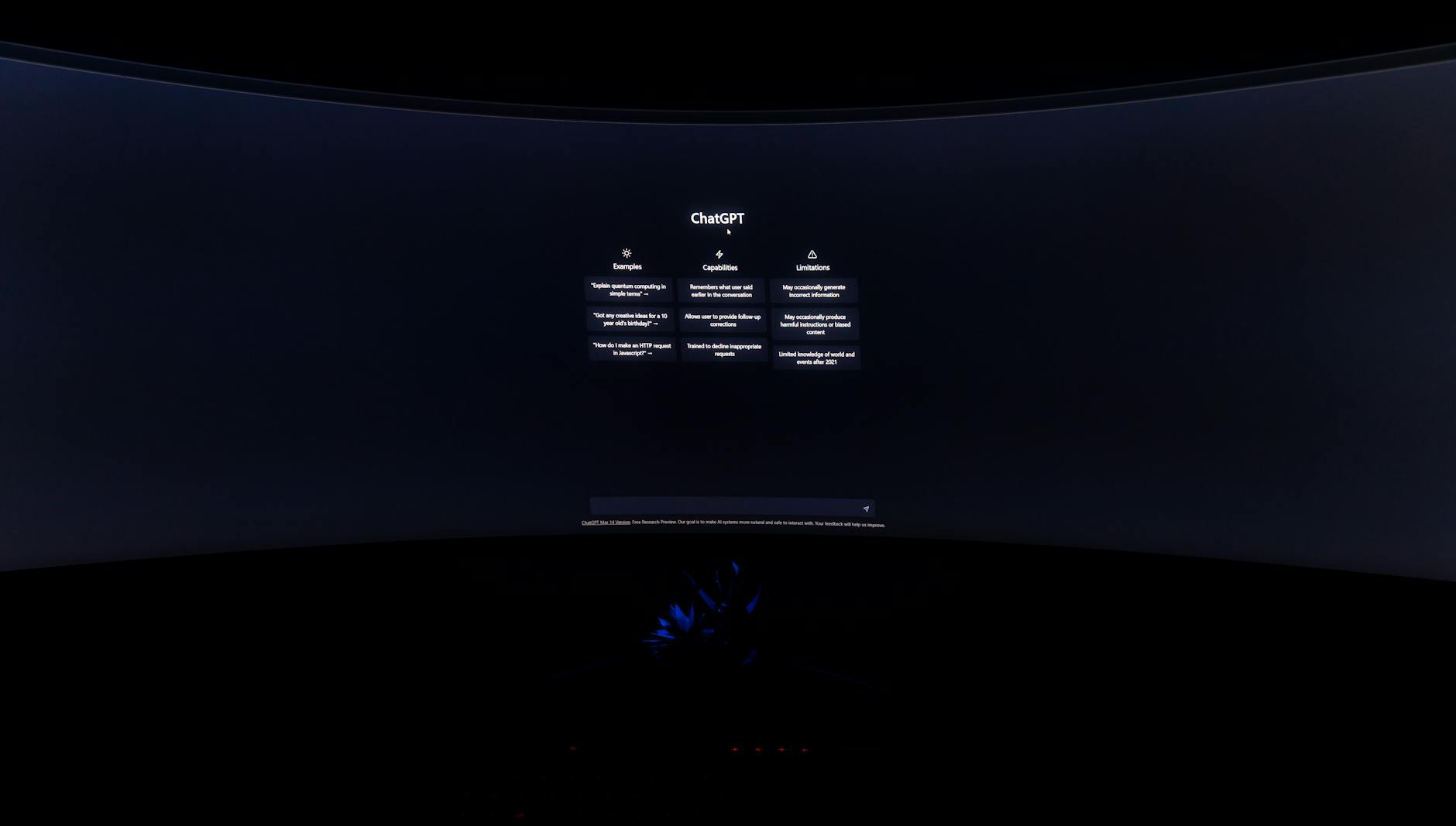
Impact on Consumer Products and Services
As the AI arms race among big tech companies intensifies, its effects are becoming increasingly visible in the realm of consumer products and services. The integration of AI technologies is transforming the way we interact with devices, consume content, manage our homes, and even approach healthcare and financial decisions. This section will explore the profound impact of AI on various aspects of our daily lives, focusing on four key areas: AI-powered virtual assistants, personalized recommendations and experiences, smart home devices and IoT integration, and AI applications in healthcare and finance.
A. AI-powered virtual assistants
AI-powered virtual assistants have become an integral part of our digital lives, revolutionizing the way we interact with technology and access information. These intelligent agents, such as Apple’s Siri, Amazon’s Alexa, Google Assistant, and Microsoft’s Cortana, are at the forefront of the AI arms race among big tech companies. As these companies vie for dominance in the virtual assistant market, consumers are reaping the benefits of increasingly sophisticated and capable AI companions.
Natural Language Processing Advancements
One of the most significant improvements in AI-powered virtual assistants has been in the realm of natural language processing (NLP). As the competition intensifies, companies are investing heavily in developing more advanced NLP algorithms, enabling their virtual assistants to understand and respond to human speech with greater accuracy and nuance.
- Contextual understanding: Modern virtual assistants can now grasp the context of conversations, allowing for more natural and fluid interactions.
- Multilingual support: Many assistants now offer support for multiple languages and dialects, breaking down language barriers for users worldwide.
- Sentiment analysis: Advanced AI algorithms can detect the user’s emotional state, allowing virtual assistants to respond with appropriate empathy and tone.
Expanded Capabilities and Integration
As the AI arms race progresses, virtual assistants are expanding their capabilities and becoming more deeply integrated into our daily routines:
- Cross-platform functionality: Virtual assistants are no longer confined to smartphones; they now operate seamlessly across various devices, including smart speakers, cars, and wearables.
- Task automation: AI-powered assistants can now handle complex, multi-step tasks, such as booking travel arrangements or scheduling appointments with minimal human intervention.
- IoT device control: Virtual assistants serve as central hubs for controlling smart home devices, allowing users to manage their connected ecosystems through voice commands.
Voice Commerce and Conversational AI
The integration of AI-powered virtual assistants with e-commerce platforms has given rise to voice commerce, a new frontier in online shopping:
- Voice-activated purchasing: Users can now make purchases, reorder items, and track shipments using voice commands alone.
- Personalized product recommendations: AI assistants leverage user data and preferences to suggest relevant products and deals.
- Conversational product search: Natural language queries enable users to find products more intuitively, without the need for precise search terms.
Privacy and Security Considerations
As virtual assistants become more prevalent and collect increasing amounts of personal data, privacy and security concerns have come to the forefront:
- Data encryption: Companies are implementing stronger encryption methods to protect user data and communications.
- Selective data retention: Some virtual assistants now offer options for users to control how long their voice data is stored or to delete it entirely.
- Transparency in data usage: Tech giants are facing pressure to be more transparent about how they use and share user data collected through virtual assistants.
The Future of AI-powered Virtual Assistants
As the AI arms race continues, we can expect to see even more advanced features and capabilities in virtual assistants:
- Emotional intelligence: Future virtual assistants may be able to recognize and respond to complex human emotions, providing more empathetic and personalized interactions.
- Proactive assistance: AI assistants could anticipate user needs and offer suggestions or take actions before being prompted.
- Augmented reality integration: Virtual assistants may leverage AR technology to provide visual overlays and guidance in real-world settings.
Feature | Current State | Future Potential |
---|---|---|
Language Understanding | Advanced NLP with contextual awareness | Near-human level comprehension of nuance and subtext |
Task Complexity | Multi-step task automation | Handling of complex, open-ended problems |
Device Integration | Cross-platform functionality | Seamless integration with all aspects of digital and physical life |
Privacy Controls | User-controlled data retention | Fully anonymized, decentralized data processing |
Emotional Intelligence | Basic sentiment analysis | Deep understanding and response to complex emotional states |
As AI-powered virtual assistants continue to evolve, they are poised to become even more central to our daily lives, serving as intelligent interfaces between us and the increasingly complex digital world.
B. Personalized recommendations and experiences
The AI arms race has significantly impacted the way companies deliver personalized recommendations and experiences to consumers. By leveraging advanced machine learning algorithms and vast amounts of user data, tech giants are continuously improving their ability to tailor content, products, and services to individual preferences. This personalization extends across various domains, including entertainment, e-commerce, news, and social media.
Content Streaming and Entertainment
The entertainment industry has been revolutionized by AI-driven personalization, with streaming platforms leading the charge:
- Recommendation engines: Services like Netflix, Spotify, and YouTube use sophisticated AI algorithms to analyze viewing and listening habits, offering highly personalized content suggestions.
- Dynamic content creation: Some platforms are experimenting with AI-generated content tailored to individual user preferences, potentially revolutionizing the entertainment industry.
- Adaptive streaming: AI optimizes video and audio quality based on device capabilities and network conditions, ensuring the best possible viewing or listening experience.
E-commerce and Product Recommendations
AI-powered personalization has transformed the online shopping experience:
- Product discovery: AI algorithms analyze browsing history, purchase patterns, and demographic data to suggest relevant products to shoppers.
- Dynamic pricing: Some e-commerce platforms use AI to adjust prices in real-time based on demand, competition, and individual user behavior.
- Visual search: AI-powered image recognition allows users to search for products using photos, enhancing the shopping experience.
News and Information Curation
The way we consume news and information has been significantly impacted by AI-driven personalization:
- Personalized news feeds: Social media platforms and news aggregators use AI to curate content based on user interests and engagement patterns.
- Topic clustering: AI algorithms group related news stories, providing users with comprehensive coverage of topics they’re interested in.
- Automated summarization: AI-powered tools can generate concise summaries of lengthy articles, catering to users’ preferences for quick information consumption.
Social Media and User-Generated Content
AI plays a crucial role in personalizing social media experiences:
- Content prioritization: Social media platforms use AI to determine which posts appear in users’ feeds, based on their interests and interactions.
- Friend and connection suggestions: AI algorithms analyze social graphs to recommend potential connections and foster community building.
- Automated content moderation: AI systems help platforms identify and filter out inappropriate or harmful content, creating safer online environments.
Challenges and Ethical Considerations
While personalization offers numerous benefits, it also raises important ethical questions and challenges:
- Filter bubbles: Highly personalized content can create echo chambers, potentially limiting exposure to diverse perspectives.
- Data privacy: The extensive data collection required for effective personalization raises concerns about user privacy and data security.
- Algorithmic bias: AI systems may inadvertently perpetuate or amplify existing biases, leading to unfair or discriminatory outcomes.
The Future of Personalized Experiences
As the AI arms race continues, we can expect even more advanced personalization techniques:
- Cross-platform personalization: AI systems may provide seamless personalized experiences across multiple devices and platforms.
- Predictive personalization: AI could anticipate user needs and preferences before they’re explicitly expressed.
- Contextual personalization: Recommendations may adapt in real-time based on factors like location, time of day, and current activities.
Personalization Domain | Current Applications | Future Potential |
---|---|---|
Entertainment | Content recommendations, adaptive streaming | AI-generated, personalized content |
E-commerce | Product suggestions, dynamic pricing | Virtual personal shoppers, AR try-on experiences |
News and Information | Personalized feeds, topic clustering | Real-time, personalized news generation |
Social Media | Content prioritization, connection suggestions | Predictive social interactions, AI-curated communities |
As AI technologies continue to advance, the line between personalized and generalized experiences will likely blur, offering users increasingly tailored interactions with digital products and services.
C. Smart home devices and IoT integration
The AI arms race has significantly accelerated the development and adoption of smart home devices and Internet of Things (IoT) integration. As tech giants compete to dominate this growing market, consumers are benefiting from increasingly sophisticated and interconnected home ecosystems. This section explores the impact of AI on smart home technology and the broader IoT landscape.
AI-powered Smart Home Hubs
Central to the smart home revolution are AI-powered hubs that serve as the brain of the connected home:
- Voice-controlled assistants: Devices like Amazon Echo, Google Home, and Apple HomePod use AI to understand and execute voice commands, controlling various smart home devices.
- Learning algorithms: These hubs employ machine learning to understand user habits and preferences, automatically adjusting settings for optimal comfort and efficiency.
- Interoperability: AI helps bridge the gap between devices from different manufacturers, enabling seamless integration and control.
Smart Energy Management
AI is revolutionizing home energy management, leading to increased efficiency and cost savings:
- Intelligent thermostats: Devices like Nest and Ecobee use AI to learn occupancy patterns and adjust temperature settings automatically.
- Smart lighting: AI-powered lighting systems adapt to natural light levels and occupancy, optimizing energy use and comfort.
- Energy consumption analysis: AI algorithms analyze household energy use, providing insights and recommendations for reducing consumption.
Advanced Home Security Systems
AI has significantly enhanced home security capabilities:
- Facial recognition: Smart doorbells and security cameras use AI to identify familiar faces and alert homeowners to potential threats.
- Anomaly detection: AI algorithms can detect unusual patterns or sounds, triggering alerts for potential security breaches.
- Predictive maintenance: AI-powered systems can anticipate equipment failures and schedule maintenance before issues occur.
IoT Device Ecosystem
The proliferation of IoT devices has created a complex ecosystem that AI helps manage and optimize:
- Device discovery and setup: AI simplifies the process of adding new devices to the home network, automatically detecting and configuring them.
- Cross-device communication: AI facilitates seamless interaction between various IoT devices, enabling complex automation scenarios.
- Data aggregation and analysis: AI systems collect and analyze data from multiple IoT devices, providing holistic insights into home operations.
Challenges and Considerations
The rapid growth of smart home and IoT technologies presents several challenges:
- Privacy concerns: The extensive data collection required for smart home operation raises questions about user privacy and data security.
- Interoperability issues: Despite efforts to create universal standards, compatibility between devices from different manufacturers remains a challenge.
- Complexity and user experience: As smart home systems become more sophisticated, ensuring a user-friendly experience becomes increasingly important.
The Future of Smart Homes and IoT
As the AI arms race continues, we can expect to see further advancements in smart home and IoT technologies:
- Predictive home management: AI systems may anticipate household needs and take proactive actions, such as ordering groceries or scheduling maintenance.
- Enhanced contextual awareness: Smart homes could adapt to individual family members’ preferences and routines, providing personalized experiences for each occupant.
- Seamless integration with wearables and vehicles: The smart home ecosystem may extend beyond the house, integrating with personal devices and smart cars for a truly connected lifestyle.
Smart Home Domain | Current Applications | Future Potential |
---|---|---|
Energy Management | Adaptive thermostats, smart lighting | Predictive energy optimization, integration with renewable sources |
Security | Facial recognition, anomaly detection | AI-powered threat assessment, autonomous security drones |
Home Automation | Voice-controlled routines, scheduled tasks | Context-aware automation, AI-generated custom routines |
Healthcare | Basic health monitoring devices | AI-powered diagnostics, personalized health recommendations |
Entertainment | Smart TVs, multi-room audio systems | Immersive AR/VR experiences, AI-curated content libraries |
As AI continues to evolve, smart homes and IoT devices will become increasingly intelligent and intuitive, transforming the way we interact with our living spaces and the objects around us.
D. AI in healthcare and finance
The AI arms race has had a profound impact on two critical sectors: healthcare and finance. As tech giants and startups compete to develop cutting-edge AI solutions, these industries are experiencing rapid transformation, leading to improved patient outcomes, more efficient financial services, and innovative approaches to longstanding challenges.
AI in Healthcare
The integration of AI in healthcare has the potential to revolutionize patient care, diagnosis, and treatment:
Medical Imaging and Diagnostics
AI is making significant strides in enhancing medical imaging and diagnostic capabilities:
- Image analysis: AI algorithms can analyze medical images such as X-rays, MRIs, and CT scans with high accuracy, often detecting abnormalities that human radiologists might miss.
- Early disease detection: Machine learning models can identify patterns in medical data that may indicate the early stages of diseases like cancer or neurological disorders.
- Diagnostic assistance: AI-powered tools help healthcare professionals make more accurate diagnoses by analyzing patient data and comparing it with vast databases of medical information.
Personalized Treatment Plans
AI is enabling the development of more personalized and effective treatment strategies:
- Genomic analysis: AI algorithms can analyze genetic data to identify potential disease risks and tailor treatment plans to individual patients.
- Drug discovery: Machine learning accelerates the drug discovery process by predicting how different compounds will interact with biological targets.
- Treatment optimization: AI systems can analyze patient data and medical literature to suggest optimal treatment plans based on individual patient characteristics.
Remote Patient Monitoring
AI-powered devices and applications are enhancing remote patient care:
- Wearable devices: AI algorithms analyze data from wearable devices to monitor patients’ vital signs and alert healthcare providers to potential issues.
- Telemedicine: AI-powered chatbots and virtual assistants facilitate remote consultations and triage patients based on symptom severity.
- Chronic disease management: AI systems help patients manage chronic conditions by providing personalized recommendations and reminders for medication and lifestyle changes.
Challenges in Healthcare AI
Despite its promise, the implementation of AI in healthcare faces several challenges:
- Data privacy and security: The sensitive nature of medical data requires robust protection measures and clear consent protocols.
- Regulatory compliance: AI systems in healthcare must meet stringent regulatory requirements to ensure patient safety and efficacy.
- Integration with existing systems: Implementing AI solutions in healthcare often requires integration with legacy systems and workflows, which can be complex and time-consuming.
AI in Finance
The financial sector has been quick to adopt AI technologies, leading to more efficient operations and innovative services:
Algorithmic Trading
AI has revolutionized trading strategies and market analysis:
- High-frequency trading: AI algorithms execute trades at speeds impossible for human traders, taking advantage of minute market fluctuations.
- Market prediction: Machine learning models analyze vast amounts of financial data to predict market trends and inform investment decisions.
- Risk assessment: AI systems evaluate complex financial instruments and market conditions to assess and manage risk more effectively.
Fraud Detection and Prevention
AI plays a crucial role in enhancing financial security:
- Transaction monitoring: Machine learning algorithms analyze transaction patterns to identify potentially fraudulent activities in real-time.
- Identity verification: AI-powered biometric systems, such as facial recognition and voice analysis, enhance security for financial transactions.
- Anti-money laundering: AI tools help financial institutions detect and prevent money laundering by identifying suspicious patterns in financial data.
Personalized Financial Services
AI is enabling more tailored and accessible financial products:
- Robo-advisors: AI-powered investment platforms provide personalized portfolio management based on individual risk profiles and financial goals.
- Credit scoring: Machine learning models analyze alternative data sources to assess creditworthiness, potentially expanding access to financial services.
- Chatbots and virtual assistants: AI-powered chatbots provide 24/7 customer support and personalized financial advice.
Challenges in Finance AI
The implementation of AI in finance also faces several challenges:
- Algorithmic bias: AI systems may perpetuate or amplify existing biases in financial decision-making, potentially leading to unfair outcomes.
- Explainability: The complexity of AI algorithms can make it difficult to explain financial decisions to regulators and customers.
- Market volatility: While AI can analyze vast amounts of data, unexpected events or “black swan” occurrences can still lead to market disruptions.
The Future of AI in Healthcare and Finance
As the AI arms race continues, we can expect to see even more advanced applications in healthcare and finance:
- Quantum computing in finance: The integration of quantum computing with AI could revolutionize complex financial modeling and risk assessment.
- AI-powered drug development: Advanced AI systems may be able to design and test new drugs entirely in silico, dramatically accelerating the development process.
- Predictive healthcare: AI could enable highly accurate predictions of individual health outcomes, allowing for truly personalized preventive care.
Domain | Current Applications | Future Potential |
---|---|---|
Healthcare Imaging | Anomaly detection in medical images | Real-time, AI-assisted surgical guidance |
Personalized Medicine | Genomic analysis for treatment planning | AI-designed, patient-specific drugs |
Financial Trading | High-frequency trading, market prediction | Quantum AI for complex market modeling |
Fraud Prevention | Transaction monitoring, identity verification | Predictive fraud detection across global financial networks |
As AI technologies continue to advance, the healthcare and finance sectors will likely see even greater transformation, leading to more personalized, efficient, and accessible services for consumers worldwide.
Now that we have explored the profound impact of AI on consumer products and services, including virtual assistants, personalized experiences, smart home devices, and applications in healthcare and finance, it’s clear that the AI
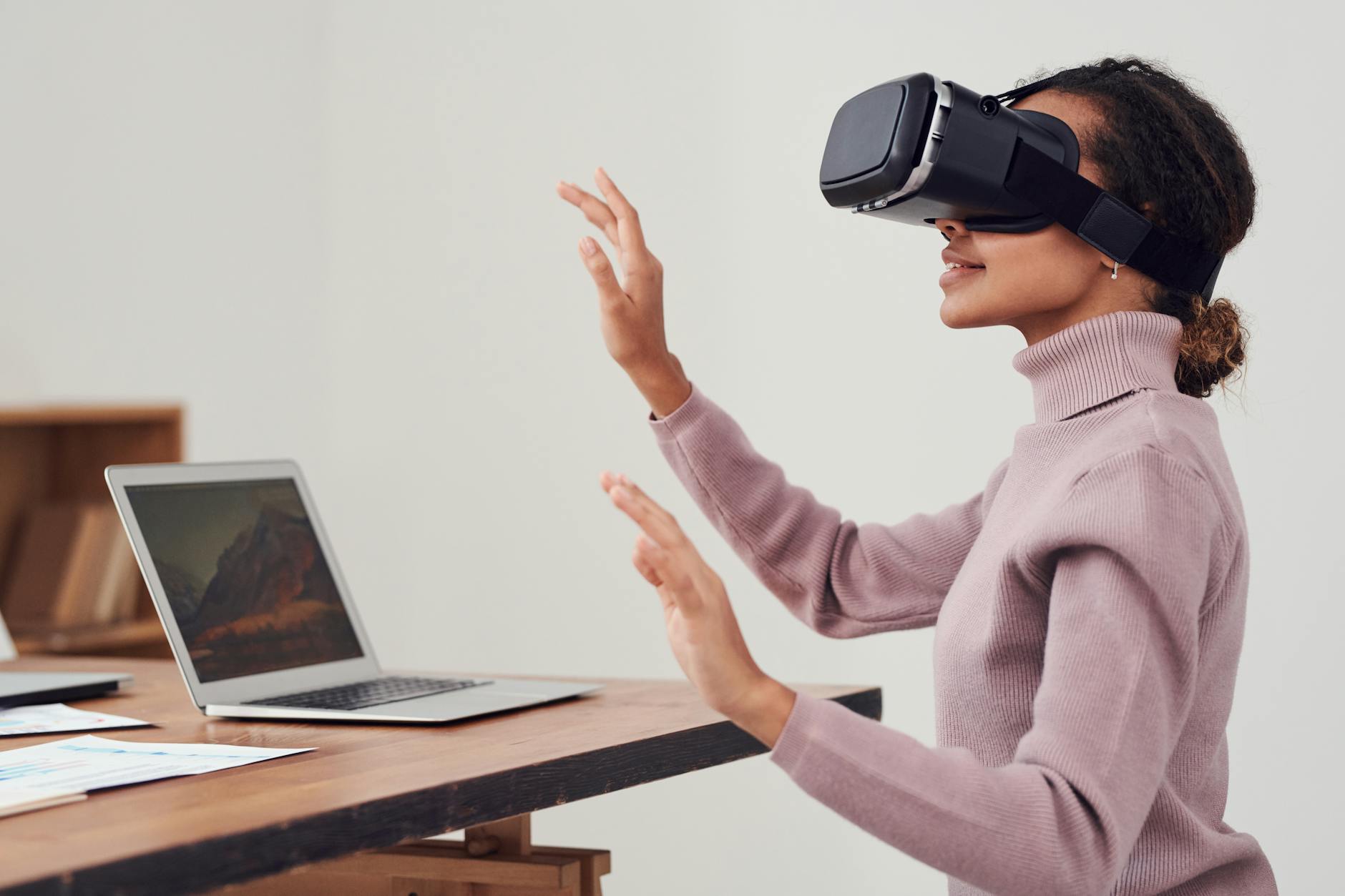
The Future of AI Competition
As we delve into the future of AI competition, it’s crucial to understand how the landscape is evolving and what implications this has for various stakeholders. The AI arms race among big tech companies is not just about who can develop the most advanced algorithms or amass the most data; it’s about shaping the future of technology, society, and the global economy. Let’s explore the potential collaborations, emerging markets, and long-term implications of this fierce competition.
A. Potential collaborations and partnerships
The future of AI competition is not solely about cutthroat rivalry; it also involves strategic alliances and collaborations. As the field becomes more complex and resource-intensive, even the biggest tech giants are realizing the value of joining forces.
1. Cross-industry collaborations
One of the most promising trends in AI development is the emergence of cross-industry collaborations. These partnerships bring together expertise from various sectors, fostering innovation and accelerating progress. For example:
- Healthcare and AI: Major tech companies are partnering with healthcare providers and pharmaceutical firms to develop AI-powered diagnostic tools, drug discovery platforms, and personalized treatment plans.
- Automotive and AI: Collaborations between car manufacturers and tech companies are driving the development of autonomous vehicles and smart transportation systems.
- Finance and AI: Banks and fintech startups are joining forces with AI companies to create more sophisticated fraud detection systems, algorithmic trading platforms, and personalized financial advice services.
These collaborations not only pool resources but also combine domain expertise with cutting-edge AI capabilities, leading to more practical and impactful solutions.
2. Academic-industry partnerships
The relationship between academia and industry is becoming increasingly important in the AI arms race. Tech giants are forging strong ties with universities and research institutions to:
- Access top talent
- Fund cutting-edge research
- Gain insights into emerging AI technologies
For instance, Google’s collaboration with Stanford University on AI ethics research or Microsoft’s partnership with MIT on AI for healthcare are prime examples of such academic-industry alliances.
These partnerships benefit both parties:
- Companies get access to groundbreaking research and a pipeline of skilled graduates
- Universities receive funding and real-world applications for their research
Benefits for Companies | Benefits for Universities |
---|---|
Access to cutting-edge research | Funding for research projects |
Talent pipeline | Real-world application of theories |
Early insights into emerging technologies | Industry exposure for students |
Influence on academic curricula | Access to industry data and resources |
3. Open-source initiatives
The future of AI competition will likely see a rise in open-source initiatives. Despite the competitive nature of the AI race, many companies are recognizing the value of open-source projects in accelerating innovation and fostering a collaborative ecosystem. Examples include:
- TensorFlow (Google)
- PyTorch (Facebook)
- BERT (Google)
- GPT (OpenAI)
These open-source tools and models allow developers worldwide to contribute to and build upon existing AI technologies, potentially leading to breakthroughs that benefit the entire industry.
4. International collaborations
As AI becomes increasingly global, we can expect to see more international collaborations. These partnerships may take various forms:
- Government-to-government collaborations on AI research and policy
- Cross-border industry partnerships
- International academic exchanges and joint research programs
Such collaborations can help address global challenges, share best practices, and ensure that AI development is not limited to a few dominant countries or companies.
5. AI consortiums and alliances
The formation of AI consortiums and alliances is another trend that’s likely to shape the future of AI competition. These groups bring together multiple stakeholders to:
- Set industry standards
- Address ethical concerns
- Pool resources for large-scale projects
Examples include:
- Partnership on AI: A consortium of tech companies, academic institutions, and non-profits working to ensure that AI benefits society.
- AI Global: An international non-profit focused on responsible AI development and deployment.
These alliances can help create a more level playing field and ensure that AI development is guided by ethical principles and societal needs.
B. Emerging AI markets and applications
As AI technology continues to advance, we’re seeing the emergence of new markets and applications that will shape the future of the industry. These emerging areas represent significant opportunities for companies in the AI race.
1. AI in edge computing
Edge computing, which involves processing data closer to its source rather than in centralized cloud servers, is becoming increasingly important in the AI landscape. This trend is driven by:
- The need for real-time processing in applications like autonomous vehicles and IoT devices
- Privacy concerns and data regulations that limit the transfer of sensitive information
- The desire to reduce latency and bandwidth usage
AI at the edge presents several opportunities:
- More efficient and responsive IoT devices
- Enhanced privacy and security for AI applications
- Reduced dependence on cloud infrastructure
Companies that can effectively integrate AI capabilities into edge devices will have a significant advantage in this emerging market.
2. AI in quantum computing
The intersection of AI and quantum computing is an area of intense research and development. Quantum computing has the potential to revolutionize AI by:
- Solving complex optimization problems much faster than classical computers
- Enhancing machine learning algorithms
- Enabling more sophisticated simulations and modeling
While still in its early stages, quantum AI could lead to breakthroughs in areas such as:
- Drug discovery
- Financial modeling
- Climate change prediction
- Cryptography
Companies that can successfully leverage quantum computing for AI applications may gain a significant competitive edge in the future.
3. AI in augmented and virtual reality
The integration of AI with augmented reality (AR) and virtual reality (VR) technologies is opening up new possibilities for immersive experiences. This combination can enhance:
- Gaming and entertainment
- Education and training
- Remote collaboration
- Design and prototyping
AI can improve AR and VR experiences by:
- Enhancing object recognition and tracking
- Providing more natural language interactions
- Personalizing content based on user behavior
- Improving rendering and graphics quality
As AR and VR technologies become more widespread, the ability to integrate sophisticated AI capabilities will be a key differentiator for companies in this space.
4. AI in bioengineering and synthetic biology
The application of AI to bioengineering and synthetic biology is an emerging field with enormous potential. AI can accelerate research and development in areas such as:
- Gene editing and therapy
- Protein folding prediction
- Synthetic organism design
- Biofuel production
Companies that can effectively apply AI to these complex biological problems may unlock new possibilities in healthcare, agriculture, and environmental sustainability.
5. AI in climate tech
As the world grapples with climate change, AI is emerging as a powerful tool for developing and implementing climate solutions. Applications include:
- Optimizing renewable energy systems
- Improving climate models and predictions
- Enhancing energy efficiency in buildings and industries
- Monitoring and reducing carbon emissions
The intersection of AI and climate tech represents a significant opportunity for companies to make a positive impact while also tapping into a growing market.
6. AI in personalized medicine
The future of healthcare is likely to be heavily influenced by AI-driven personalized medicine. This emerging field combines AI with genomics, wearable technology, and big data analytics to provide tailored healthcare solutions. Applications include:
- Personalized treatment plans based on genetic profiles
- Early disease detection through AI analysis of medical imaging
- AI-powered virtual health assistants
- Precision drug dosing
Companies that can effectively leverage AI in personalized medicine may revolutionize healthcare delivery and outcomes.
C. Long-term implications for society and economy
The AI arms race among big tech companies will have far-reaching consequences for society and the global economy. Understanding these long-term implications is crucial for policymakers, business leaders, and the general public.
1. Economic transformation
AI is poised to fundamentally transform the global economy in several ways:
a. Job market disruption
One of the most significant impacts of AI will be on the job market. While AI will create new job opportunities, it will also lead to the automation of many existing roles. This shift will likely result in:
- Increased demand for AI specialists, data scientists, and other tech-related roles
- Decline in repetitive and routine jobs across various sectors
- Need for continuous upskilling and reskilling of the workforce
To mitigate the negative impacts, societies will need to:
- Invest in education and training programs to prepare workers for AI-driven economies
- Develop policies to support workers during the transition
- Explore new economic models, such as universal basic income, to address potential job losses
b. Productivity gains
AI has the potential to significantly boost productivity across various sectors of the economy. This could lead to:
- Increased economic output and GDP growth
- More efficient resource allocation
- Lower costs for goods and services
However, the distribution of these productivity gains will be a crucial societal challenge. Ensuring that the benefits of AI-driven productivity are shared equitably will be essential for maintaining social stability.
c. New business models and industries
The AI arms race will likely give rise to entirely new business models and industries. Examples might include:
- AI-as-a-Service platforms
- Personal AI assistants
- AI-driven content creation and curation services
- AI ethics consultancies
These new industries will create economic opportunities but may also disrupt existing business models and market structures.
d. Shift in global economic power
The outcomes of the AI arms race could potentially shift the balance of global economic power. Countries and companies that successfully leverage AI may gain significant competitive advantages, potentially altering the current economic world order.
2. Social and cultural impacts
The widespread adoption of AI will have profound social and cultural implications:
a. Changes in human interaction
As AI becomes more integrated into our daily lives, it may significantly alter how we interact with each other and with technology. This could lead to:
- Increased reliance on AI for decision-making and problem-solving
- Changes in social norms and communication patterns
- Potential isolation or decreased human-to-human interaction in some areas
b. Privacy and surveillance concerns
The data-hungry nature of AI systems raises significant privacy concerns. As AI becomes more pervasive, we may see:
- Increased surveillance capabilities
- Challenges to personal privacy and data ownership
- Need for new legal and ethical frameworks to protect individual rights
c. Impact on education and learning
AI will likely transform education and learning processes:
- Personalized learning experiences tailored to individual needs
- AI-powered tutoring and assessment systems
- Shift in focus from memorization to critical thinking and creativity
d. Changes in entertainment and media consumption
AI is already changing how we consume and create media:
- AI-generated content (music, art, writing)
- Hyper-personalized entertainment recommendations
- Virtual and augmented reality experiences enhanced by AI
These changes may have profound effects on cultural production and consumption.
3. Ethical and philosophical considerations
The AI arms race raises numerous ethical and philosophical questions that society will need to grapple with:
a. AI rights and consciousness
As AI systems become more sophisticated, questions about AI rights and consciousness may arise:
- Should highly advanced AI systems have legal rights?
- How do we define and measure AI consciousness?
- What are the ethical implications of creating potentially sentient AI?
b. Human-AI relationships
The nature of human-AI relationships will likely evolve, raising questions such as:
- What are the boundaries of appropriate human-AI interaction?
- How will AI companions affect human relationships and emotional well-being?
- What are the psychological impacts of increased human-AI interaction?
c. AI decision-making and accountability
As AI systems take on more decision-making roles, issues of accountability and transparency become crucial:
- Who is responsible when AI systems make mistakes or cause harm?
- How can we ensure transparency in AI decision-making processes?
- What role should AI play in high-stakes decisions (e.g., healthcare, criminal justice)?
d. AI and human values
Ensuring that AI systems align with human values and ethics is a significant challenge:
- How can we encode human values into AI systems?
- Who decides which values should be prioritized?
- How do we address cultural differences in values when developing global AI systems?
4. Geopolitical implications
The AI arms race will have significant geopolitical implications:
a. National security and military applications
AI is becoming increasingly important in national security and military contexts:
- AI-powered cybersecurity systems
- Autonomous weapons and defense systems
- AI-enhanced intelligence gathering and analysis
The development of these technologies could alter the balance of military power and create new security challenges.
b. AI governance and regulation
As AI becomes more powerful and pervasive, international cooperation on AI governance will be crucial:
- Development of global AI standards and norms
- Regulation of AI development and deployment
- International agreements on AI ethics and safety
c. AI-driven diplomacy and international relations
AI could transform how nations interact and conduct diplomacy:
- AI-powered analysis of international relations
- Use of AI in treaty negotiations and conflict resolution
- Potential for AI to mediate international disputes
d. Technological sovereignty
The AI arms race may lead to increased focus on technological sovereignty:
- Nations striving for independence in AI development
- Restrictions on cross-border data flows and AI technologies
- Competition for control of key AI resources (e.g., data, computing power)
5. Environmental impacts
The environmental implications of the AI arms race are significant and multifaceted:
a. Energy consumption
The training and operation of large AI models require substantial computational resources, leading to:
- Increased energy consumption and associated carbon emissions
- Need for more energy-efficient AI algorithms and hardware
- Potential strain on power grids and energy resources
b. AI for environmental solutions
On the positive side, AI can be a powerful tool for addressing environmental challenges:
- Optimizing energy grids for renewable integration
- Improving climate models and predictions
- Enhancing wildlife conservation efforts
- Developing more sustainable manufacturing processes
c. E-waste and hardware lifecycle
The rapid advancement of AI technologies may lead to increased e-waste:
- Frequent upgrades of AI-capable devices
- Obsolescence of older hardware
- Need for better recycling and circular economy practices in the tech industry
d. Resource extraction
The demand for raw materials needed in AI hardware (e.g., rare earth elements) may lead to:
- Increased mining and resource extraction
- Potential environmental degradation in resource-rich areas
- Geopolitical tensions over access to these resources
6. Healthcare transformation
AI has the potential to revolutionize healthcare in numerous ways:
a. Personalized medicine
AI-driven personalized medicine could lead to:
- More effective and targeted treatments
- Earlier disease detection and prevention
- Reduced healthcare costs through more efficient treatment plans
b. Medical research and drug discovery
AI can accelerate medical research and drug discovery:
- Faster identification of potential drug candidates
- More efficient clinical trial processes
- Better understanding of complex diseases and biological processes
c. Healthcare delivery
AI could transform how healthcare is delivered:
- AI-powered diagnostic tools and decision support systems
- Telemedicine and remote patient monitoring
- Automated administrative tasks, freeing up healthcare workers
d. Mental health support
AI applications in mental health could provide:
- 24/7 access to mental health support through chatbots and virtual therapists
- Early detection of mental health issues through behavioral analysis
- Personalized mental health interventions and treatment plans
7. Future of work and education
The AI arms race will significantly impact the future of work and education:
a. Lifelong learning
The rapid pace of AI development will necessitate continuous learning:
- Increased emphasis on adaptability and learning new skills
- Growth of online and AI-powered learning platforms
- Shift towards modular and skill-based education models
b. Human-AI collaboration
The future workplace will likely involve close collaboration between humans and AI:
- AI augmenting human capabilities rather than replacing them entirely
- Need for new skills in managing and working alongside AI systems
- Redefinition of job roles and organizational structures
c. Remote and flexible work
AI technologies could further enable remote and flexible work arrangements:
- AI-powered collaboration tools and virtual workspaces
- Increased automation of office tasks
- Potential for 24/7 operations through human-AI teams across time zones
d. Reskilling and upskilling
As AI transforms various industries, there will be a growing need for reskilling and upskilling programs:
- Government and corporate initiatives to retrain workers
- Growth of the edtech industry
- Emphasis on developing uniquely human skills (creativity, emotional intelligence, complex problem-solving)
In conclusion, the future of AI competition will have far-reaching implications across all aspects of society and the global economy. While the potential benefits are immense, so too are the challenges and risks. As we move forward, it will be crucial for all stakeholders – from tech companies and governments to academics and citizens – to work together to ensure that the development and deployment of AI technologies serve the greater good of humanity. The decisions we make today in shaping the AI landscape will have profound effects on future generations, making it imperative that we approach this technological revolution with wisdom, foresight, and a commitment to ethical and sustainable progress.
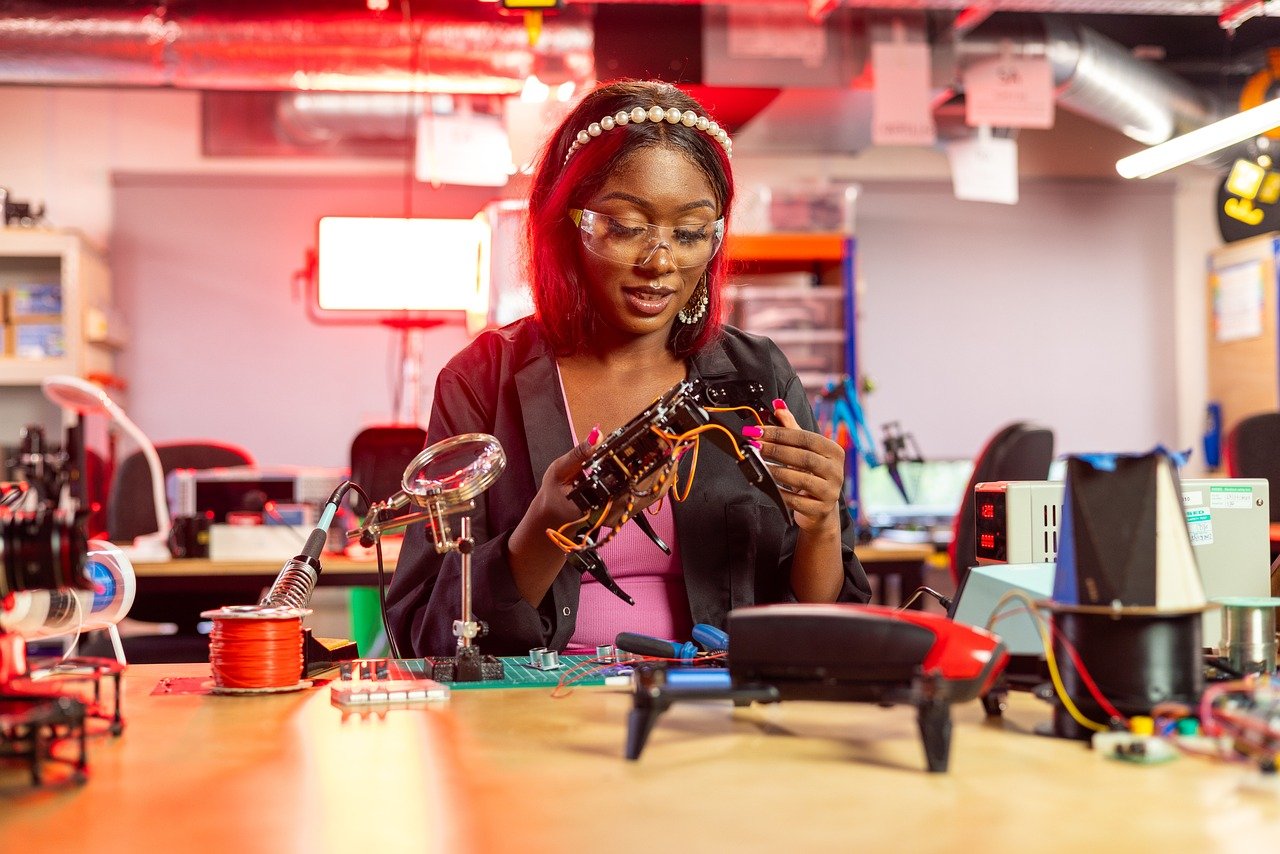
The AI arms race among tech giants has intensified, with major players like Google, Microsoft, and Meta vying for dominance in key technologies such as machine learning, natural language processing, and computer vision. As these companies compete for top AI talent and invest heavily in research and development, we’re witnessing rapid advancements in consumer products and services. However, this race also raises important ethical concerns and regulatory challenges that must be addressed.
As the AI landscape continues to evolve, it’s crucial for consumers, businesses, and policymakers to stay informed about the latest developments and their potential impacts. By understanding the competitive dynamics and technological advancements in the AI field, we can better prepare for the opportunities and challenges that lie ahead. Ultimately, responsible innovation and collaboration will be key to harnessing the full potential of AI while mitigating its risks.